Fall detection method and system based on wearable sensor and video monitoring
A technology for video surveillance and detection methods, applied in the fields of signal processing and deep learning, can solve the problems of detecting personnel falling, not processing sensor data at the same time, etc., achieving the effect of fast running speed, low overall equipment cost, and low memory usage
- Summary
- Abstract
- Description
- Claims
- Application Information
AI Technical Summary
Problems solved by technology
Method used
Image
Examples
Embodiment 1
[0046] A fall detection method based on wearable sensors and video surveillance consists of cascading two independent models, such as figure 1 As shown, they are respectively based on the Gated Recurrent Unit (Gated Recurrent Unit, GRU) sensor data analysis one-stage GRU model, and the two-stage TSM surveillance video processing model based on the time shift module TSM surveillance video analysis; in the first stage In the GRU model, GRU is a variant of LSTM, which can maintain comparable performance to LSTM while reducing the amount of calculation. In the two-stage TSM surveillance video processing model, an adaptive channel shift strategy is added to the time shift module TSM combined with channel attention, and the improved TSM is inserted into the improved feature combined with the convolutional block attention mechanism The extraction network CSPDarknet53 is used to improve the accuracy and robustness of video detection.
[0047] A fall detection method based on wearable...
Embodiment 2
[0055] According to a kind of fall detection method based on wearable sensor and video monitoring described in embodiment 1, its difference is:
[0056] In step 2, start the first-stage algorithm of fall detection based on GRU for analysis, output the result in a probabilistic manner, and initially judge whether a fall behavior occurs, specifically refers to: preprocessing the posture parameters to be detected obtained in step 1 (according to step (3 ) preprocessing method) and then input the trained one-stage GRU model for detection, wherein, the trained one-stage GRU model construction and training process are as follows:
[0057] (1) Build a one-stage GRU model: a one-stage GRU model includes multi-layer GRU, and each layer of GRU adopts 100 memory units; in the selection of the model, the present invention considers that this is the first-stage algorithm, and it is necessary to ensure a low amount of calculation At the same time, to ensure good accuracy, a multi-layer GRU ...
Embodiment 3
[0072] According to a kind of fall detection method based on wearable sensor and video monitoring described in embodiment 1, its difference is:
[0073] In step 4, use the two-stage TSM surveillance video analysis algorithm to judge whether a fall occurs, specifically refers to: input the trained two-stage TSM after preprocessing the surveillance video to be detected (according to the preprocessing method of step c) The monitoring video processing model is used for detection. Among them, the trained two-stage TSM monitoring video processing model construction and training process are as follows:
[0074] a. Construct a two-stage TSM surveillance video processing model:
[0075] like Figure 4As shown, the two-stage TSM surveillance video processing model includes a series of CMBL and CSP1-X. Through a series of CMBL operations and CSP1-X operations of different sizes, the spatio-temporal features are extracted repeatedly, and the feature map is pooled by SPP spatial pyramid p...
PUM
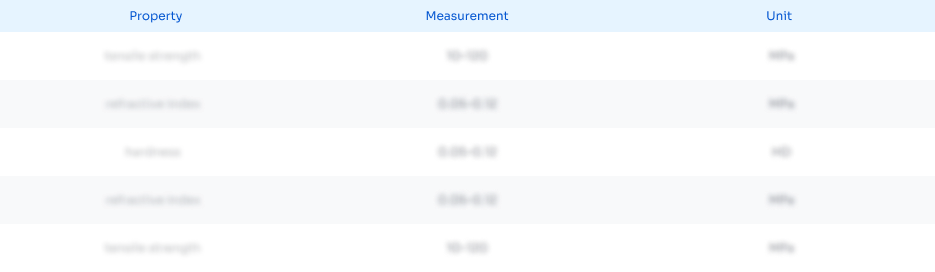
Abstract
Description
Claims
Application Information
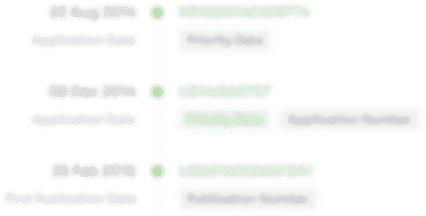
- R&D
- Intellectual Property
- Life Sciences
- Materials
- Tech Scout
- Unparalleled Data Quality
- Higher Quality Content
- 60% Fewer Hallucinations
Browse by: Latest US Patents, China's latest patents, Technical Efficacy Thesaurus, Application Domain, Technology Topic, Popular Technical Reports.
© 2025 PatSnap. All rights reserved.Legal|Privacy policy|Modern Slavery Act Transparency Statement|Sitemap|About US| Contact US: help@patsnap.com