Gear case fault detection method based on deep transfer learning
A technology of transfer learning and fault detection, applied in the direction of neural learning methods, biological neural network models, instruments, etc., can solve problems such as complex, compound faults, single fault diagnosis categories, etc., and achieve the effect of high-precision diagnosis
- Summary
- Abstract
- Description
- Claims
- Application Information
AI Technical Summary
Problems solved by technology
Method used
Examples
Embodiment Construction
[0023] Hereinafter, the present invention will be described with reference to examples, but the present invention is not limited to the following examples.
[0024] A gearbox fault detection method based on deep transfer learning, firstly collect the original signal data of the gearbox, including vibration signal, temperature signal and oil signal; use SVD matrix decomposition noise reduction to reduce the noise of the original vibration signal and remove the noise signal, retain effective vibration signals, and convert all effective vibration signals into vibration image data for backup; data cleaning of collected temperature signals and oil signals, data cleaning includes abnormal value processing, deletion of duplicate values and null value processing At least one of temperature image data and oil image data is obtained, and the temperature signal includes at least one of staged temperature rise, same-side temperature difference, staged uninterrupted temperature rise, and ...
PUM
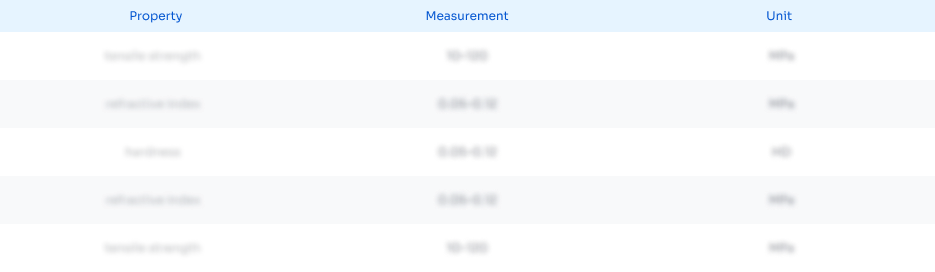
Abstract
Description
Claims
Application Information
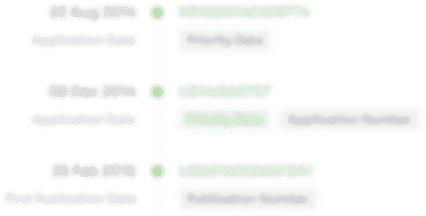
- R&D Engineer
- R&D Manager
- IP Professional
- Industry Leading Data Capabilities
- Powerful AI technology
- Patent DNA Extraction
Browse by: Latest US Patents, China's latest patents, Technical Efficacy Thesaurus, Application Domain, Technology Topic, Popular Technical Reports.
© 2024 PatSnap. All rights reserved.Legal|Privacy policy|Modern Slavery Act Transparency Statement|Sitemap|About US| Contact US: help@patsnap.com