Microbial marker for echinococcosis as well as screening method and application of microbial marker
A technology of echinococcosis and screening method, which is applied in the field of disease diagnosis, can solve the problems of high false positive rate, lack of specificity, and inability to realize early diagnosis, and achieve the effect of good specificity and high prediction sensitivity
- Summary
- Abstract
- Description
- Claims
- Application Information
AI Technical Summary
Problems solved by technology
Method used
Image
Examples
Embodiment 1
[0054] Collection of clinical stool samples and acquisition of fungal DNA data
[0055] (1) The stool samples of 63 patients with echinococcosis and 42 healthy controls were collected from the hospital, and DNA was extracted from the stool samples of patients with echinococcosis and healthy controls using a kit for extracting fecal fungal DNA, and then the ITS1 region Perform next-generation sequencing to obtain ITS1 sequencing data of intestinal flora;
[0056] (2) Search and match the Unite database (https: / / unite.ut.ee) with the BLAST method according to the ITS1 data to obtain the abundance value of each OTU. This match found 12472 OTU fungi;
Embodiment 2
[0058] Model algorithm selection and parameter optimization
[0059] The present invention obtains optimal random forest model (Random Forest) through a large amount of screening and matching, and specific method is as follows:
[0060] Use machine learning to pick model algorithms. Random forests utilize multiple decision tree classifiers whose output class is determined by the mode of the class output by individual trees. The cases of the sample data of the present invention have been clinically diagnosed and have classified labels, so they will be explored and selected in a supervised machine learning classification model. The fungal OTU abundance values of all samples were used as input data, and the diagnostic results of samples were used as output classification labels. Algorithm construction with the following steps:
[0061] (1) All data obtained in Example 1 are randomly divided into 70% training set (n=73) and 30% test set (n=32);
[0062] (2) Build a machine l...
Embodiment 3
[0066] Fungal OTU selection and parameter optimization for specific species
[0067] (1) Calculate the importance (Mean DecreaseAccuracy) score of the training set variable features obtained by the random forest model (see figure 2 ), sort from high to low according to the score.
[0068] Using the rfcv and repeat functions in the randomForest software package, using ten-fold cross-validation (repeated 5 times), calculate the average error rate of gradually increasing the number of fungal variables (sorted according to the importance of variables) (see image 3 ), to find the number of fungal OTUs with the smallest error rate. The results showed that the error rate was the lowest when inputting the fungal abundance of 32 specific species as the characteristic variable.
[0069] (2) Input the OTU abundance values of 32 specific species in the sample, construct a random forest model, and perform a grid search (grid) to optimize the mtry parameter using the ten-fold cross-va...
PUM
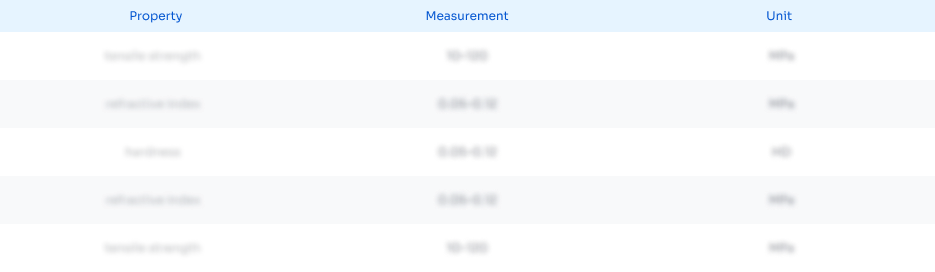
Abstract
Description
Claims
Application Information
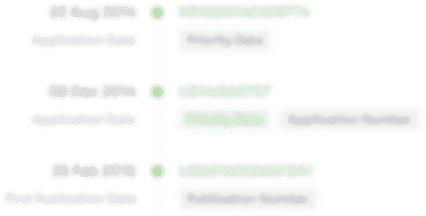
- R&D Engineer
- R&D Manager
- IP Professional
- Industry Leading Data Capabilities
- Powerful AI technology
- Patent DNA Extraction
Browse by: Latest US Patents, China's latest patents, Technical Efficacy Thesaurus, Application Domain, Technology Topic.
© 2024 PatSnap. All rights reserved.Legal|Privacy policy|Modern Slavery Act Transparency Statement|Sitemap