Method for classifying electroencephalogram signals based on variable parameter recurrent neural network
A recurrent neural network and EEG technology, applied in the field of EEG signal classification based on variable-parameter recurrent neural network, can solve problems such as slow solution speed, achieve high robustness, improve signal-to-noise ratio, classification accuracy and high precision effect
- Summary
- Abstract
- Description
- Claims
- Application Information
AI Technical Summary
Problems solved by technology
Method used
Image
Examples
Embodiment 1
[0089] figure 1 This embodiment is a flow chart of a method for classifying EEG signals based on variable-parameter recurrent neural network. The illustrated steps can complete the classification of EEG signals based on wavelet transform support vector recurrent neural network.
[0090] A method and system implementation of EEG signal classification based on variable-parameter recurrent neural network, comprising the following steps:
[0091] 1) Collect the user's EEG signal through the electrode cap, and then preprocess the data, including the determination of the time window, the selection of electrode channels, continuous wavelet transform and normalization;
[0092] 2) Based on the preprocessed data in step 1), using the principle of soft margin support vector machine, construct the convex quadratic programming problem of the motor imagery EEG signal classifier;
[0093] 3) Introduce the convex quadratic programming problem in step 2) into a penalty function to eliminate ...
Embodiment 2
[0163] Prompt the user to imagine a certain action (imagine the left foot and imagining the right foot) through the computer display screen, and then use the 3-channel electrode cap to collect the EEG signal 3-9s after the user accepts the prompt, and then preprocess the data, including determining The time window size of data sample collection is 3.25-6.25s, the electrode channel selection channel C3, channel C4, continuous wavelet transform and normalization processing. Among them, the continuous wavelet transform uses Morlet wavelet, which is expressed as:
[0164]
[0165] where exp( ) represents exponential function, t represents time, and then continuous wavelet transform is performed on the data, which can be expressed as:
[0166]
[0167] Where s is a scaling unit, s is selected from 7-30Hz, which is used to scale the wavelet, τ represents the center frequency, and x(t) is the input signal. Then use the max-min normalization method, which is expressed as:
[0...
Embodiment 3
[0227] Prompt the user to imagine a certain action (imagine the left foot and imagining the right foot) through the computer screen, and then use the Figure 4 The 22-channel electrode cap collects EEG signals 3-9s after the user accepts the prompt, and then preprocesses the data, including determining the time window size of data sample collection 3.25-6.25s, electrode channels, continuous wavelet transform and normalization processing . Among them, the continuous wavelet transform uses Morlet wavelet, which is expressed as:
[0228]
[0229] where exp( ) represents exponential function, t represents time, and then continuous wavelet transform is performed on the data, which can be expressed as:
[0230]
[0231] Where s is a scaling unit, s is selected from 7-30Hz, which is used to scale the wavelet, τ represents the center frequency, and x(t) is the input signal. Then use the max-min normalization method, which is expressed as:
[0232]
[0233] Where x represen...
PUM
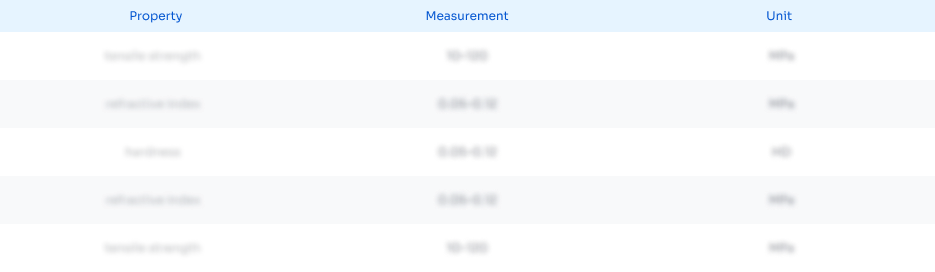
Abstract
Description
Claims
Application Information
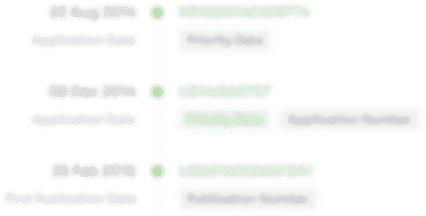
- R&D Engineer
- R&D Manager
- IP Professional
- Industry Leading Data Capabilities
- Powerful AI technology
- Patent DNA Extraction
Browse by: Latest US Patents, China's latest patents, Technical Efficacy Thesaurus, Application Domain, Technology Topic.
© 2024 PatSnap. All rights reserved.Legal|Privacy policy|Modern Slavery Act Transparency Statement|Sitemap