Method for predicting remaining service life of battery based on maximum expectation-unscented particle filter
An unscented particle filter and maximum expectation technology, applied in interdisciplinary fields, can solve problems such as battery capacity increase, battery remaining service life prediction error, etc., and achieve the effect of reducing prediction error
- Summary
- Abstract
- Description
- Claims
- Application Information
AI Technical Summary
Problems solved by technology
Method used
Image
Examples
specific Embodiment approach 1
[0036] Embodiment 1: The specific process of the battery remaining service life prediction method based on the maximum expectation-unscented particle filter in this embodiment is as follows:
[0037] Step 1. Extract the battery capacity data in the kth working process;
[0038] Extracting the capacity data of the battery in the kth actual working cycle, that is, the charge and discharge test can be carried out after each working cycle of the battery, and the battery capacity data under the corresponding cycle can be obtained as the input of the battery remaining service life prediction method.
[0039]Step 2. According to the battery capacity data in the k-th working process extracted in step 1, construct a dynamic battery degradation model based on unscented particle filtering, which is used to describe the degradation process of the battery;
[0040] Step 3. Estimate the process noise and measurement noise in the battery degradation model adaptively by using a maximum expect...
specific Embodiment approach 2
[0047] Embodiment 2: The difference between this embodiment and Embodiment 1 is that in step 2, a dynamic battery degradation model based on unscented particle filtering is constructed according to the battery capacity data extracted in step 1 during the k-th working process , which is used to describe the degradation process of the battery; the specific process is:
[0048] Compared with the Kalman filter algorithm and the extended Kalman filter algorithm, the advantage of particle filter is that it can deal with the problem of parameter identification of nonlinear and non-Gaussian systems. It should be noted that the traditional particle filter algorithm directly uses the prior probability density function as the importance function. However, the particles generated from the prior distribution do not necessarily reflect the true particle distribution, and the measurement information at the current moment of the system is ignored. To this end, the present invention considers...
specific Embodiment approach 3
[0118] Embodiment 3: The difference between this embodiment and Embodiment 1 or 2 is that in step 3, a maximum expectation-based algorithm is used to adaptively estimate the process noise and measurement noise in the battery degradation model;
[0119] Specifically, for the process noise and measurement noise in the dynamic degradation model based on unscented particle filtering, the maximum expectation algorithm is used for adaptive estimation. recursive update;
[0120] The specific process is:
[0121] The unknown parameter vector formed by process noise and measurement noise can be expressed as:
[0122] The essence of the EM algorithm is to maximize the joint likelihood function p(x under the premise of the posterior probability. 0:k ,y 1:k |Ξ) to achieve maximum likelihood estimation of unknown parameters Ξ. Known battery capacity data y from initial to k-th duty cycle 1:k =[y 1 ,y2,…,y k ], the joint log-likelihood function L can be constructed k (Ξ) is shown ...
PUM
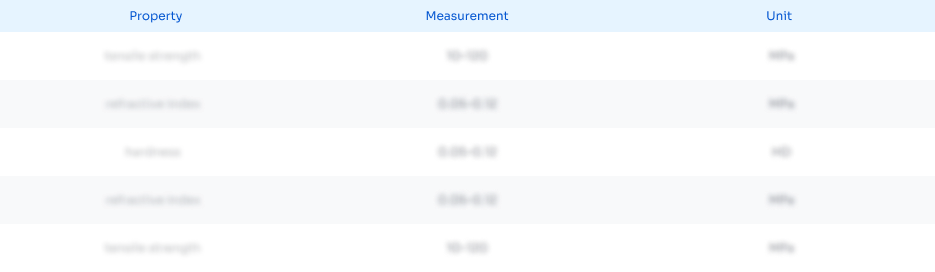
Abstract
Description
Claims
Application Information
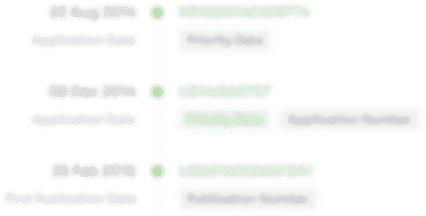
- Generate Ideas
- Intellectual Property
- Life Sciences
- Materials
- Tech Scout
- Unparalleled Data Quality
- Higher Quality Content
- 60% Fewer Hallucinations
Browse by: Latest US Patents, China's latest patents, Technical Efficacy Thesaurus, Application Domain, Technology Topic, Popular Technical Reports.
© 2025 PatSnap. All rights reserved.Legal|Privacy policy|Modern Slavery Act Transparency Statement|Sitemap|About US| Contact US: help@patsnap.com