Differential privacy federal learning method for resisting member reasoning attack
A differential privacy and learning method technology, applied in the field of machine learning, can solve the problems of reducing the performance of the global network model, poor performance of federated learning, and obstacles to federated learning training, so as to reduce the problem of insufficient training, increase performance, and improve performance.
- Summary
- Abstract
- Description
- Claims
- Application Information
AI Technical Summary
Problems solved by technology
Method used
Image
Examples
Embodiment
[0039] This embodiment takes centralized federated learning as the basic structure and trains a classifier network (global network model) based on the mnist data set as an example to illustrate the specific implementation measures of the method:
[0040] For the client, the adversarial generative network is trained using local data (mnist data), followed by generating fake data (fake mnist data) through the adversarial generative network using random noise. In the training of the federated learning mnist classifier network, the clients participating in this round of communication can use a certain amount of fake data to join the real data to participate in the training of the mnist classifier network issued by the server, or they can completely use the fake data to participate in the training of the mnist classifier issued by the server. mnist classifier network training, an alternative to the unimproved federated learning approach where clients are trained on real data. The a...
PUM
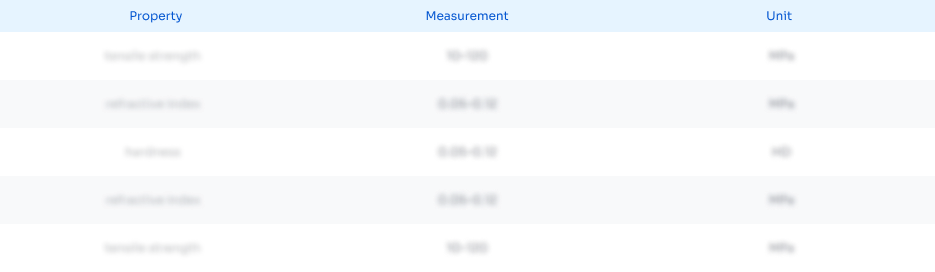
Abstract
Description
Claims
Application Information
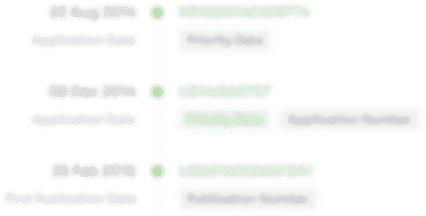
- R&D Engineer
- R&D Manager
- IP Professional
- Industry Leading Data Capabilities
- Powerful AI technology
- Patent DNA Extraction
Browse by: Latest US Patents, China's latest patents, Technical Efficacy Thesaurus, Application Domain, Technology Topic, Popular Technical Reports.
© 2024 PatSnap. All rights reserved.Legal|Privacy policy|Modern Slavery Act Transparency Statement|Sitemap|About US| Contact US: help@patsnap.com