Anticancer peptide prediction method based on bidirectional long-short-term memory network and feature fusion
A long-term and short-term memory, feature fusion technology, applied in neural learning methods, biological neural network models, for analyzing two-dimensional or three-dimensional molecular structures, etc., can solve problems such as expensive, complex, time-consuming, etc. rate effect
- Summary
- Abstract
- Description
- Claims
- Application Information
AI Technical Summary
Problems solved by technology
Method used
Image
Examples
Embodiment Construction
[0055] The present invention will be further described below with reference to the accompanying drawings and embodiments. This figure is a simplified schematic diagram, and only illustrates the basic structure of the present invention in a schematic manner, so it only shows the structure related to the present invention.
[0056] like figure 1 As shown, the anticancer peptide prediction method based on bidirectional long short-term memory network and feature fusion includes the following steps:
[0057]Step 1. Read the four benchmark peptide sequence data sets, and analyze the amino acid composition of the data sets. The data sets are in Table 1. The data set analysis is as follows image 3 shown;
[0058] Table 1 Four benchmark peptide sequence datasets
[0059]
[0060]
[0061] Amino acid alphabet coding The primary letter sequence of the peptide is numerically encoded, that is, the 20 basic amino acids are assigned numbers 1-20, and the peptide sequences with insuf...
PUM
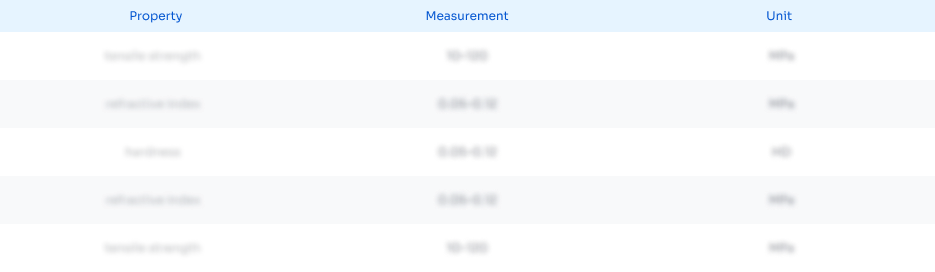
Abstract
Description
Claims
Application Information
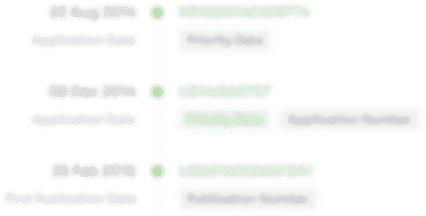
- R&D Engineer
- R&D Manager
- IP Professional
- Industry Leading Data Capabilities
- Powerful AI technology
- Patent DNA Extraction
Browse by: Latest US Patents, China's latest patents, Technical Efficacy Thesaurus, Application Domain, Technology Topic, Popular Technical Reports.
© 2024 PatSnap. All rights reserved.Legal|Privacy policy|Modern Slavery Act Transparency Statement|Sitemap|About US| Contact US: help@patsnap.com