Vehicle model identification method based on pooling multi-scale depth convolution characteristics
A technology of deep convolution and car model recognition, applied in character and pattern recognition, biological neural network model, image data processing, etc., can solve poor performance, lack of geometric invariance of convolutional neural network, limited variable scene classification and Matching and other issues, to achieve the effect of simple and clear thinking, reduced memory consumption, high practicability and robustness
- Summary
- Abstract
- Description
- Claims
- Application Information
AI Technical Summary
Problems solved by technology
Method used
Image
Examples
Embodiment Construction
[0042] In order to describe the technical content, structural features, achieved goals and effects of the present invention in detail, the following will be described in detail in conjunction with the embodiments and accompanying drawings.
[0043] The invention proposes a vehicle type recognition method based on pooled multi-scale deep convolution features, which achieves good results in vehicle type recognition. The schematic diagram of the whole algorithm is shown in figure 1 shown, including steps:
[0044] Step 1: For each car model image in the car model image database, extract its deep convolution features according to different scales, and scale 1 is not processed;
[0045] Specifically, for each car model image, the depth convolution features at three scales are extracted here, and no further operation is performed on scale 1, only the depth convolution features of the remaining two scales are processed, including the following steps:
[0046] Step 1.1: Obtain the m...
PUM
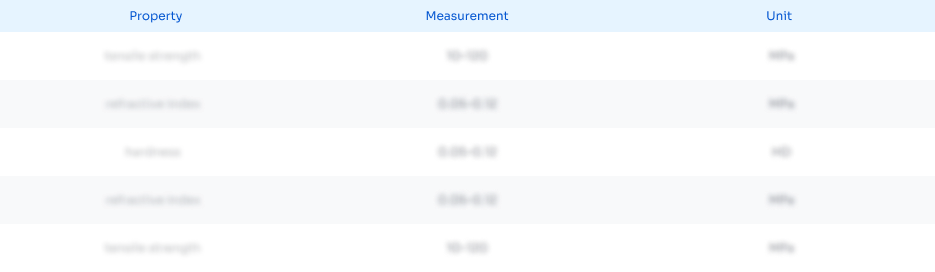
Abstract
Description
Claims
Application Information
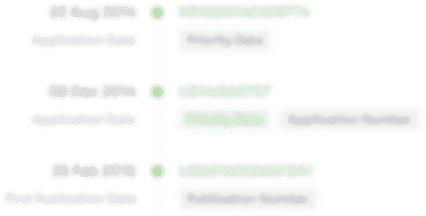
- R&D Engineer
- R&D Manager
- IP Professional
- Industry Leading Data Capabilities
- Powerful AI technology
- Patent DNA Extraction
Browse by: Latest US Patents, China's latest patents, Technical Efficacy Thesaurus, Application Domain, Technology Topic, Popular Technical Reports.
© 2024 PatSnap. All rights reserved.Legal|Privacy policy|Modern Slavery Act Transparency Statement|Sitemap|About US| Contact US: help@patsnap.com