Unbalanced data classification method based on adaptive upsampling
A classification method and upsampling technology, applied in the field of pattern recognition, can solve problems such as unsatisfactory effects, achieve the effect of enhancing learning and improving overall performance
- Summary
- Abstract
- Description
- Claims
- Application Information
AI Technical Summary
Problems solved by technology
Method used
Image
Examples
Embodiment Construction
[0017] The present invention is subject to adaptive upsampling algorithm and figure 1 Inspired by the Adaboost algorithm shown, the two are combined to form an ensemble classifier. The present invention will be described in further detail below in conjunction with the accompanying drawings.
[0018] (1) Obtaining test and training data: the present invention selects the vehicle type identification database in the KEEL database, which contains 846 samples altogether. The positive samples in the database are small truck data, a total of 199, namely n p =199. Negative samples include the data of three types of vehicles including buses, Opel cars, and Saab cars, a total of 647, that is, n n =647. The database contains a total of 18-dimensional features such as torque, steering radius, and maximum braking distance. Calculate the unbalance rate according to formula (1),
[0019] IR=n n / n p (1)
[0020] It can be obtained that the imbalance ratio in this experiment should b...
PUM
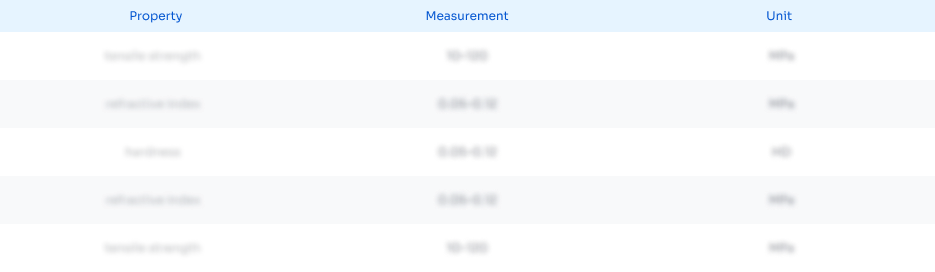
Abstract
Description
Claims
Application Information
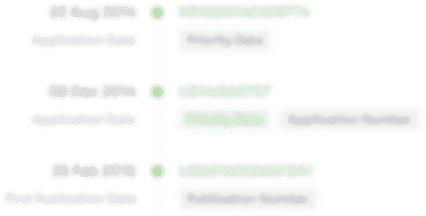
- R&D
- Intellectual Property
- Life Sciences
- Materials
- Tech Scout
- Unparalleled Data Quality
- Higher Quality Content
- 60% Fewer Hallucinations
Browse by: Latest US Patents, China's latest patents, Technical Efficacy Thesaurus, Application Domain, Technology Topic, Popular Technical Reports.
© 2025 PatSnap. All rights reserved.Legal|Privacy policy|Modern Slavery Act Transparency Statement|Sitemap|About US| Contact US: help@patsnap.com