Parallelization method of convolutional neural networks in fuzzy region under big-data environment
A convolutional neural network, fuzzy area technology, applied in biological neural network model, neural architecture, electrical digital data processing and other directions, can solve problems such as low utilization rate, lack of big data processing platform, waste of resources, etc.
- Summary
- Abstract
- Description
- Claims
- Application Information
AI Technical Summary
Problems solved by technology
Method used
Image
Examples
Embodiment Construction
[0040] The following will clearly and completely describe the technical solutions in the embodiments of the present invention with reference to the accompanying drawings in the embodiments of the present invention. Obviously, the described embodiments are only some, not all, embodiments of the present invention. Based on the embodiments of the present invention, all other embodiments obtained by persons of ordinary skill in the art without making creative efforts belong to the protection scope of the present invention.
[0041] Well logging data is characterized by ambiguity, and there are many reasons for this ambiguity, including the data space pollution of well logging data caused by noise, inconsistency, incompleteness, etc. The systematic data differences brought about by these problems and the ambiguity of logging data caused by these problems all restrict the accurate identification of logging facies.
[0042] The present invention proposes a parallelization method of f...
PUM
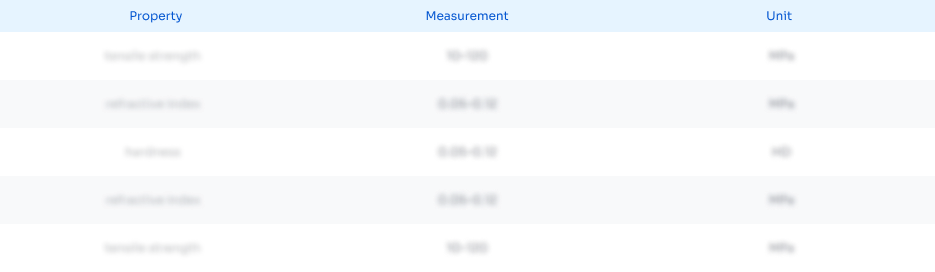
Abstract
Description
Claims
Application Information
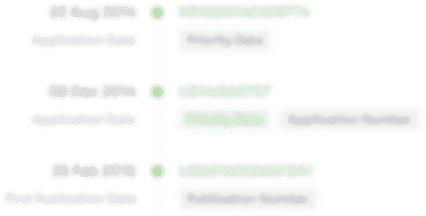
- R&D
- Intellectual Property
- Life Sciences
- Materials
- Tech Scout
- Unparalleled Data Quality
- Higher Quality Content
- 60% Fewer Hallucinations
Browse by: Latest US Patents, China's latest patents, Technical Efficacy Thesaurus, Application Domain, Technology Topic, Popular Technical Reports.
© 2025 PatSnap. All rights reserved.Legal|Privacy policy|Modern Slavery Act Transparency Statement|Sitemap|About US| Contact US: help@patsnap.com