Method for predicting power consumption of cement production which implies deep belief network of time series
A deep belief network and time series technology, applied in the field of power consumption prediction of cement production, can solve the problems of artificial neural network difficult to solve the time-varying delay, difficult to find the change law of big data, and unable to solve the time-varying delay, etc. Remove the data preprocessing process, avoid limitations, and improve the effect of convergence speed
- Summary
- Abstract
- Description
- Claims
- Application Information
AI Technical Summary
Problems solved by technology
Method used
Image
Examples
Embodiment Construction
[0022] Below in conjunction with accompanying drawing, the present invention is described in further detail:
[0023] The invention proposes a cement power consumption forecasting method with an implicit time series deep belief network. Firstly, variable selection is carried out, and the training sample set and forecast sample set are selected from the energy management system database of the cement enterprise; then, the input layer of the deep belief network is reconstructed and the cement energy consumption prediction model of the implicit time series deep belief network is completed. Its structure is as follows: figure 1 Shown; The implicit time series deep belief network cement power consumption forecasting system flow diagram that the present invention proposes is as Figure 4 Shown; Finally, the BP algorithm is used to conduct supervised global reverse fine-tuning, correct the error and optimize the weight and bias, and complete the construction of the HTS-DBN prediction...
PUM
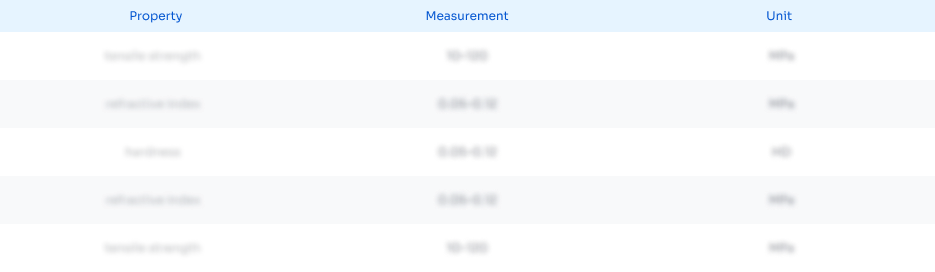
Abstract
Description
Claims
Application Information
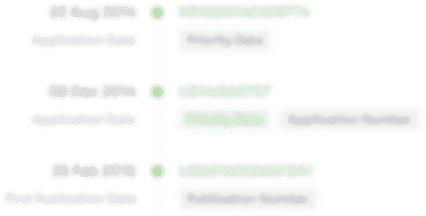
- R&D Engineer
- R&D Manager
- IP Professional
- Industry Leading Data Capabilities
- Powerful AI technology
- Patent DNA Extraction
Browse by: Latest US Patents, China's latest patents, Technical Efficacy Thesaurus, Application Domain, Technology Topic, Popular Technical Reports.
© 2024 PatSnap. All rights reserved.Legal|Privacy policy|Modern Slavery Act Transparency Statement|Sitemap|About US| Contact US: help@patsnap.com