A three-dimensional segmentation method of brain MRI hippocampus based on depth learning
A technology of deep learning and hippocampus, applied in the fields of machine learning and computer vision, can solve problems such as low contrast, unclear boundaries, and difficult to accurately segment, and achieve the effect of shortening the segmentation time, increasing the richness, and high segmentation accuracy
- Summary
- Abstract
- Description
- Claims
- Application Information
AI Technical Summary
Problems solved by technology
Method used
Image
Examples
Embodiment Construction
[0046] Specific embodiments of the present invention will be described in detail below in combination with technical solutions and accompanying drawings.
[0047] Such as figure 2 As shown, the network structure of the present invention mainly combines FCN, U-Net 3D and convolutional neural network CNN.
[0048] U-Net is a semantic segmentation network based on FCN. In the U-Net structure, down-sampling and up-sampling are combined, bottom-level information and high-level information are combined, and the bottom-level features (same resolution cascade) are used to improve the lack of up-sampling information. Significantly improve the accuracy of segmentation. However, medical image data is generally less, and the underlying features are still important. Compared with ordinary images, medical images have very high complexity, large gray scale range, and unclear boundaries, so the U-Net structure is very suitable. U-Net technology is used for medical image segmentation, such ...
PUM
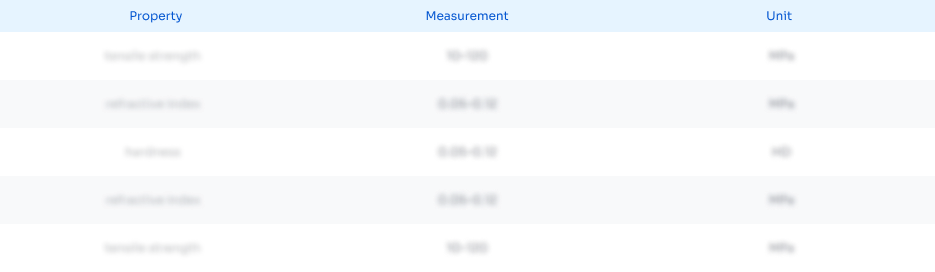
Abstract
Description
Claims
Application Information
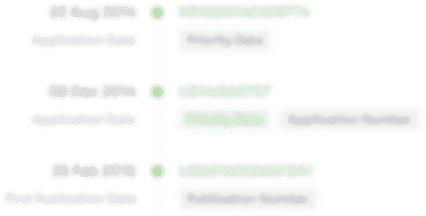
- R&D
- Intellectual Property
- Life Sciences
- Materials
- Tech Scout
- Unparalleled Data Quality
- Higher Quality Content
- 60% Fewer Hallucinations
Browse by: Latest US Patents, China's latest patents, Technical Efficacy Thesaurus, Application Domain, Technology Topic, Popular Technical Reports.
© 2025 PatSnap. All rights reserved.Legal|Privacy policy|Modern Slavery Act Transparency Statement|Sitemap|About US| Contact US: help@patsnap.com