Defect detection method for irregular metal machining surface based on depth learning
A technology of deep learning and defect detection, which is applied in graphics and image conversion, image data processing, instruments, etc., can solve the problems that are not suitable for the construction of real-time detection system, fail to meet the requirements, and have many detection stations, and achieve enhanced robustness. Stickiness and generalization ability, increase running speed, improve the effect of detection rate
- Summary
- Abstract
- Description
- Claims
- Application Information
AI Technical Summary
Problems solved by technology
Method used
Image
Examples
Embodiment
[0044] The present invention relates to a method for detecting defects on irregularly shaped metal processing surfaces based on deep learning, such as figure 1 As shown, the method includes the following steps:
[0045] Step 1: Collect the original image of the irregularly shaped metal processing surface.
[0046] Step 2: Preprocess the collected original image to obtain the ROI, and cut the ROI into square subimages of the same size to meet the input requirements of the deep learning network.
[0047] The collected images are reflected images under the light source at the same angle, but because the contour and shape of the metal surface to be tested are different, it sometimes includes some information other than the surface to be tested. Therefore, it is necessary to preprocess the original image, highlight the feature detection area and filter out the interference area, and normalize the scale of the processed image to unify the input image of the deep network. On the on...
PUM
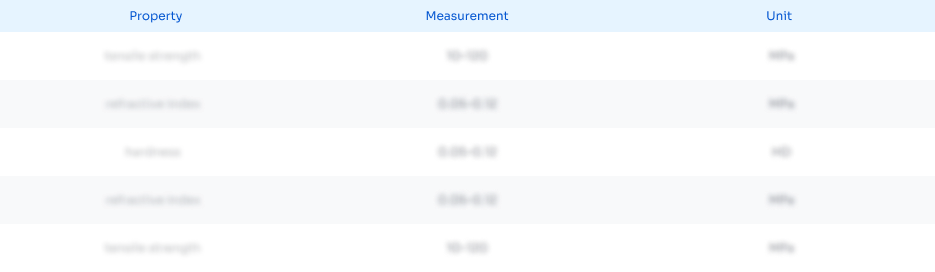
Abstract
Description
Claims
Application Information
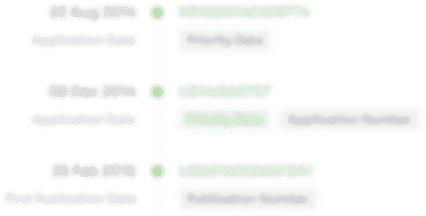
- R&D Engineer
- R&D Manager
- IP Professional
- Industry Leading Data Capabilities
- Powerful AI technology
- Patent DNA Extraction
Browse by: Latest US Patents, China's latest patents, Technical Efficacy Thesaurus, Application Domain, Technology Topic, Popular Technical Reports.
© 2024 PatSnap. All rights reserved.Legal|Privacy policy|Modern Slavery Act Transparency Statement|Sitemap|About US| Contact US: help@patsnap.com