Dailyload curve dimensionality reduction clustering method based on kernel principal component analysis
A technology of nuclear principal component analysis and clustering method, which is applied in the direction of instruments, character and pattern recognition, data processing applications, etc., and can solve problems such as weight determination of dimensionality reduction indicators
- Summary
- Abstract
- Description
- Claims
- Application Information
AI Technical Summary
Problems solved by technology
Method used
Image
Examples
Embodiment Construction
[0078] The daily load curve dimensionality reduction clustering method based on nuclear principal component analysis proposed by the present invention is described in detail as follows in conjunction with the accompanying drawings:
[0079] The general train of thought block diagram of the present invention is as figure 1 shown, including the following steps:
[0080] 1) Preprocess the daily load power curve data to obtain the original data matrix A∈R N×m . Among them, N is the number of daily load curves, and m is the dimension, that is, the number of sampling points;
[0081] 2) In combination with the original data matrix A obtained in step 1), a Gaussian radial basis (RBF) kernel function is selected for nonlinear mapping to a high-dimensional feature space to obtain a kernel matrix K and correct it;
[0082] 3) Perform principal component analysis on the revised kernel matrix K to determine the principal component components and the number of dimensionality reduction i...
PUM
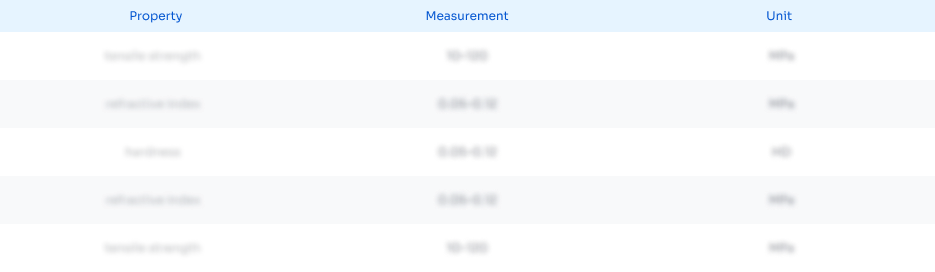
Abstract
Description
Claims
Application Information
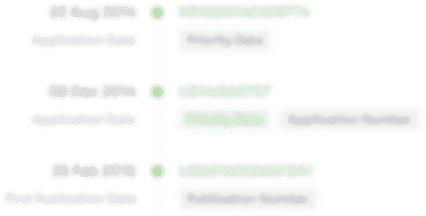
- R&D
- Intellectual Property
- Life Sciences
- Materials
- Tech Scout
- Unparalleled Data Quality
- Higher Quality Content
- 60% Fewer Hallucinations
Browse by: Latest US Patents, China's latest patents, Technical Efficacy Thesaurus, Application Domain, Technology Topic, Popular Technical Reports.
© 2025 PatSnap. All rights reserved.Legal|Privacy policy|Modern Slavery Act Transparency Statement|Sitemap|About US| Contact US: help@patsnap.com