Convolutional neural network automatic segmentation method and system for mammary molybdenum target data set
A convolutional neural network and convolutional neural technology, applied in the field of automatic segmentation and system of convolutional neural network for mammography data set, can solve the problems of squeezing the learning ability of deep learning model, troubled landing, model time-consuming, etc. Achieve the effect of improving test speed, ensuring accuracy and improving practicability
- Summary
- Abstract
- Description
- Claims
- Application Information
AI Technical Summary
Problems solved by technology
Method used
Image
Examples
Embodiment 1
[0055] This embodiment provides a convolutional neural network automatic segmentation method applied to a small breast mammography data set, which realizes the automatic segmentation of a deep convolutional neural network in a breast mammography data set, and utilizes a knowledge distillation method based on attention transfer, While retaining the characteristics of the domain, the large model trained on the big data is compressed into a small model, and then fine-tuned on the small data set, so as to ensure the accuracy of the model on the small data set and greatly reduce the parameters of the model.
[0056] Please refer to the attached figure 1 , a convolutional neural network automatic segmentation method applied to a small mammography data set proposed in this embodiment includes the following steps:
[0057] S101, selecting a large convolutional neural network to pre-train it in a large breast mammography data set.
[0058] Specifically, in the step 101, a fully convol...
Embodiment 2
[0108] This embodiment provides a convolutional neural network automatic segmentation system applied to a small breast mammography data set, the system comprising:
[0109] The model training module is configured to pre-train the large convolutional neural network on the mammography large data set;
[0110] The model compression module is configured to perform model compression on the trained large convolutional neural network by using attention transfer and knowledge distillation methods to obtain a small convolutional neural network;
[0111] A model fine-tuning module configured to fine-tune a small convolutional neural network on a small mammography dataset.
[0112] In this embodiment, the model training module is specifically configured as:
[0113] Construct a large-scale mammography data set;
[0114] Select a fully convolutional neural network;
[0115] On the large breast mammography data set, the convolutional neural network is pre-trained through the backpropaga...
PUM
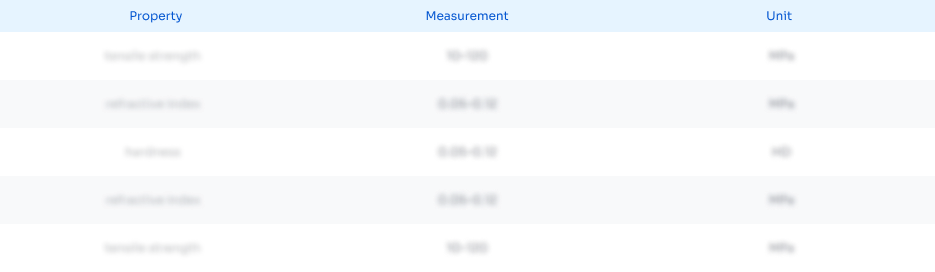
Abstract
Description
Claims
Application Information
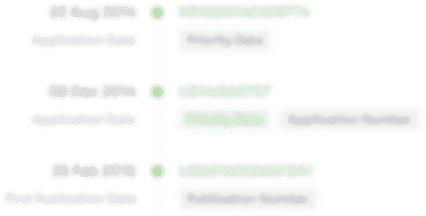
- R&D Engineer
- R&D Manager
- IP Professional
- Industry Leading Data Capabilities
- Powerful AI technology
- Patent DNA Extraction
Browse by: Latest US Patents, China's latest patents, Technical Efficacy Thesaurus, Application Domain, Technology Topic, Popular Technical Reports.
© 2024 PatSnap. All rights reserved.Legal|Privacy policy|Modern Slavery Act Transparency Statement|Sitemap|About US| Contact US: help@patsnap.com