Residuum hydrogenation model based on semi-supervised deep GRU and establishing method thereof
A technology for residual oil hydrogenation and method establishment, which is applied in character and pattern recognition, instruments, computer components, etc., can solve problems such as inability to nonlinearly fit chemical processes, gradient disappearance, and low utilization rate, so as to improve data utilization rate and information mining, improve accuracy and robustness, and accelerate the effect of secondary training speed
- Summary
- Abstract
- Description
- Claims
- Application Information
AI Technical Summary
Problems solved by technology
Method used
Image
Examples
Embodiment Construction
[0035] The present invention is described in further detail with reference to the accompanying drawings and specific embodiments.
[0036] figure 1 It is a flowchart of a method for establishing a residual oil hydrogenation model based on a semi-supervised depth GRU of the present invention.
[0037] In this specific embodiment, the method of the present invention is verified by using the production data sample of the residual oil hydrogenation unit. The data set contains 1153 pieces of measurement data with a sampling interval of 10 minutes, including 7 process variables and the new hydrogen flow rate to be predicted.
[0038] For the production data sample of the residual oil hydrogenation unit, the steps of implementing the method for establishing the residual oil hydrogenation model based on the semi-supervised depth GRU proposed by the present invention are as follows:
[0039] Step (1), for a sample set with 1153 samples and 7 independent variables, define sample set X...
PUM
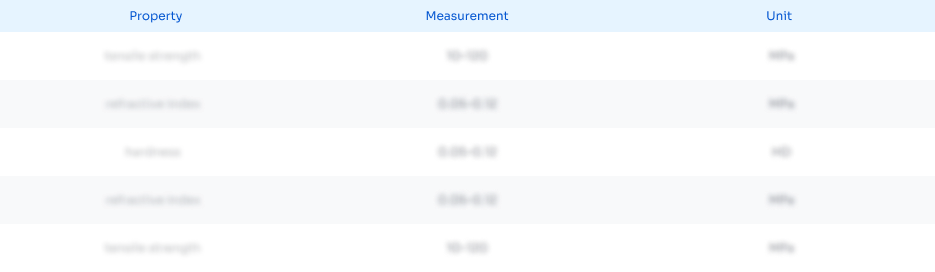
Abstract
Description
Claims
Application Information
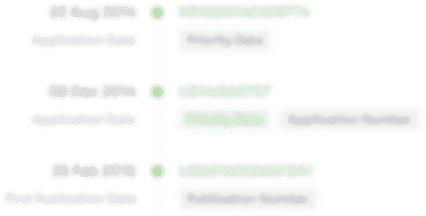
- R&D Engineer
- R&D Manager
- IP Professional
- Industry Leading Data Capabilities
- Powerful AI technology
- Patent DNA Extraction
Browse by: Latest US Patents, China's latest patents, Technical Efficacy Thesaurus, Application Domain, Technology Topic, Popular Technical Reports.
© 2024 PatSnap. All rights reserved.Legal|Privacy policy|Modern Slavery Act Transparency Statement|Sitemap|About US| Contact US: help@patsnap.com