A Deep Learning Approach to Computational Lithography
A deep learning and computational lithography technology, applied in optics, microlithography exposure equipment, optomechanical equipment, etc., can solve problems such as limiting the scope of application, avoid training errors or training failures, simplify the training process, and improve efficiency. Effect
- Summary
- Abstract
- Description
- Claims
- Application Information
AI Technical Summary
Problems solved by technology
Method used
Image
Examples
Embodiment Construction
[0032] The present invention will be described in detail below with reference to the accompanying drawings and examples.
[0033] Please refer to figure 1 , figure 1 A deep learning method for computational lithography provided by an embodiment of the present invention is shown, and its basic scheme is:
[0034] S1. Based on the lithographic imaging model, construct the mask graphic update formula in the gradient iterative algorithm; expand the iterative process of the gradient iterative algorithm, intercept the first K steps of iterations, and use each step of the iterative process as a layer in MCNN to create a K layer feed-forward convolutional neural network. The present invention refers to this model-based convolutional neural network as a model-driven convolutional neural network MCNN. The feed-forward MCNN can be considered as an encoder.
[0035] The input of the encoder is a given ideal circuit layout, and its output is the corresponding OPC mask pattern. Here, "...
PUM
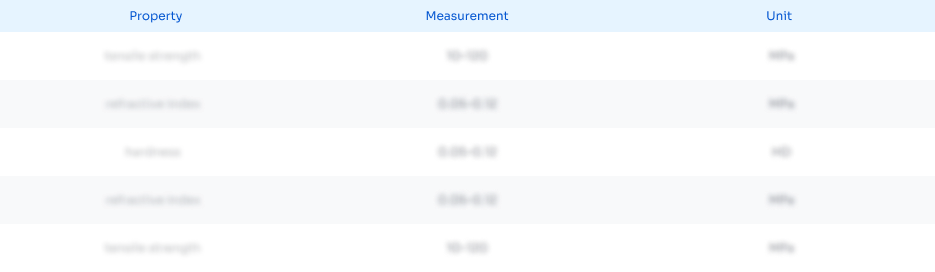
Abstract
Description
Claims
Application Information
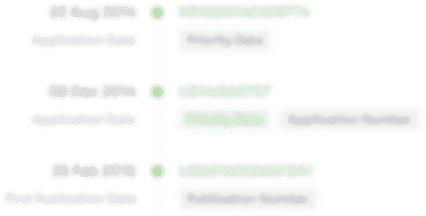
- R&D Engineer
- R&D Manager
- IP Professional
- Industry Leading Data Capabilities
- Powerful AI technology
- Patent DNA Extraction
Browse by: Latest US Patents, China's latest patents, Technical Efficacy Thesaurus, Application Domain, Technology Topic, Popular Technical Reports.
© 2024 PatSnap. All rights reserved.Legal|Privacy policy|Modern Slavery Act Transparency Statement|Sitemap|About US| Contact US: help@patsnap.com