Aerial power component image classification method based on knowledge transfer learning
A technology of transfer learning and classification method, which is applied in the field of classification of aerial photography power component images based on knowledge transfer learning, can solve the problems of huge amount of data, complex background of aerial photography power component images, and indeterminate shooting angles, etc., to improve the ability of expression and classification Good effect, ensure the effect of safe operation
- Summary
- Abstract
- Description
- Claims
- Application Information
AI Technical Summary
Problems solved by technology
Method used
Image
Examples
Embodiment Construction
[0019] Aerial photography of power transmission lines by drones is used to inspect the lines. While improving the efficiency of inspections, a large number of images of power components will also be collected. For traditional digital image processing algorithms, the classification and detection effect of different power components is not good for factors such as complex background, many types of power components, complex shooting environment, unfixed shooting angle, and mutual adhesion between different types of power components. , Weak applicability. The invention makes full use of the advantages of the convolutional neural network in deep learning, utilizes the characteristics of transfer learning, and utilizes the successful experience of the classic convolutional neural network to classify the collected images of electric components. First establish a sample library of power component images, then use migration learning to create a GoogLeNet-based convolutional neural netw...
PUM
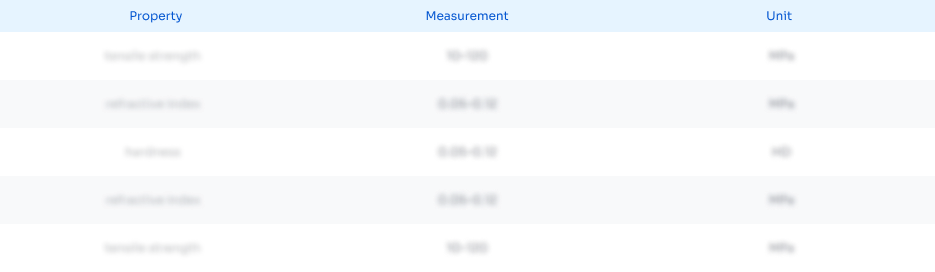
Abstract
Description
Claims
Application Information
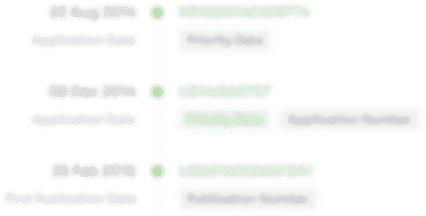
- R&D Engineer
- R&D Manager
- IP Professional
- Industry Leading Data Capabilities
- Powerful AI technology
- Patent DNA Extraction
Browse by: Latest US Patents, China's latest patents, Technical Efficacy Thesaurus, Application Domain, Technology Topic, Popular Technical Reports.
© 2024 PatSnap. All rights reserved.Legal|Privacy policy|Modern Slavery Act Transparency Statement|Sitemap|About US| Contact US: help@patsnap.com