Space-time traffic flow prediction method driven by enhanced hierarchical learning
A prediction method and technology of traffic flow, applied in traffic flow detection, traffic control system of road vehicles, traffic control system, etc., can solve the problems of delayed prediction results, low accuracy and reliability of traffic flow prediction, and redundancy.
- Summary
- Abstract
- Description
- Claims
- Application Information
AI Technical Summary
Problems solved by technology
Method used
Image
Examples
Embodiment Construction
[0062] The technical solution of a space-time traffic flow prediction method driven by enhanced hierarchical learning provided by the present invention will be further described below in conjunction with the accompanying drawings, so that those skilled in the art can better understand the present invention and implement it.
[0063] see figure 1 , a spatio-temporal traffic flow prediction method driven by enhanced hierarchical learning provided by the present invention, including extracting input data, extracting traffic flow characteristics driven by a restricted Boltzmann machine model, predicting road traffic flow based on an SVM model, and extracting input data Including highly correlated road selection extraction and original data compression based on principal component analysis, the specific steps are:
[0064] The first step is to select and extract highly connected roads;
[0065] The second step is the original data compression based on principal component analysis;...
PUM
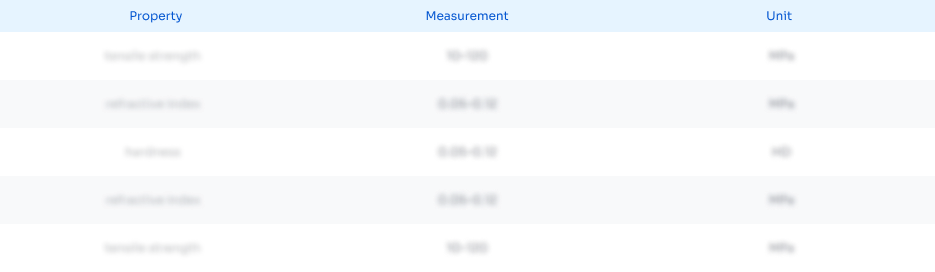
Abstract
Description
Claims
Application Information
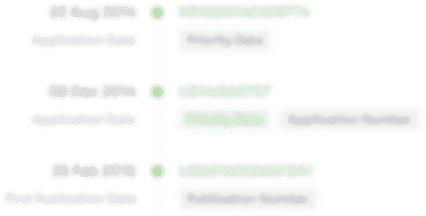
- R&D Engineer
- R&D Manager
- IP Professional
- Industry Leading Data Capabilities
- Powerful AI technology
- Patent DNA Extraction
Browse by: Latest US Patents, China's latest patents, Technical Efficacy Thesaurus, Application Domain, Technology Topic, Popular Technical Reports.
© 2024 PatSnap. All rights reserved.Legal|Privacy policy|Modern Slavery Act Transparency Statement|Sitemap|About US| Contact US: help@patsnap.com