Enhanced low-rank sparse decomposition model medical CT image denoising method
A sparse decomposition and CT image technology, applied in the field of medical image denoising, can solve the problems of poor reconstruction effect of denoised images, loss of image edge information, etc.
- Summary
- Abstract
- Description
- Claims
- Application Information
AI Technical Summary
Problems solved by technology
Method used
Image
Examples
Embodiment 1
[0141] In this embodiment, the LIDC / IDRI lung CT image database is selected as the image data source. Collect the image data in the lung CT image database (the image size is 512×512), represented by D=L+S, where D∈Rm×n represents the unprocessed original image data, L∈R m×n Represents the low-rank image data after denoising, S∈R m×n Represents sparse noisy data.
[0142] Step 1: For medical CT original image D ∈ R m×n Perform noise estimation, and according to the calculated noise intensity σ n size, traverse the original image, perform non-locally similar block matching, and divide the original image into multiple image block matrices D composed of non-locally similar blocks j :
[0143] Step 1.1: Get the original image D ∈ R m×n , its rank is recorded as r D , choose t=3r D / 5. Perform noise estimation on the image D and calculate the noise intensity σ n ;
[0144] Step 1.1.1: Perform a singular value decomposition operation on the image D, and calculate the averag...
PUM
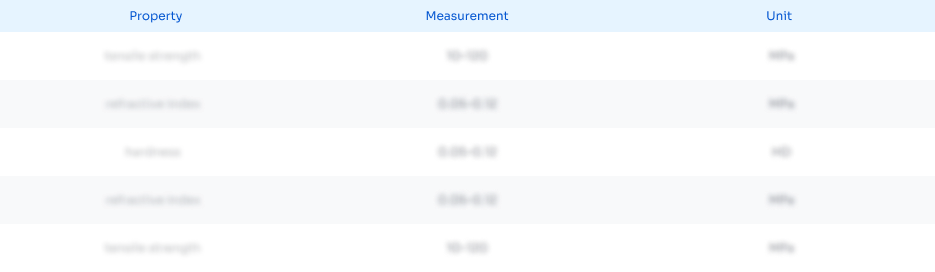
Abstract
Description
Claims
Application Information
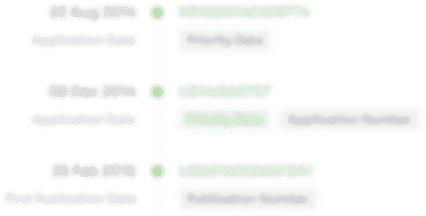
- R&D Engineer
- R&D Manager
- IP Professional
- Industry Leading Data Capabilities
- Powerful AI technology
- Patent DNA Extraction
Browse by: Latest US Patents, China's latest patents, Technical Efficacy Thesaurus, Application Domain, Technology Topic, Popular Technical Reports.
© 2024 PatSnap. All rights reserved.Legal|Privacy policy|Modern Slavery Act Transparency Statement|Sitemap|About US| Contact US: help@patsnap.com