Improved DME edema area neural network segmentation model construction method
A neural network and segmentation model technology, which is applied in the field of neural network segmentation model construction in DME edema area, can solve problems affecting the calculation accuracy of the segmentation model, focusing on pathological classification, increasing neural network training time, etc.
- Summary
- Abstract
- Description
- Claims
- Application Information
AI Technical Summary
Problems solved by technology
Method used
Image
Examples
Embodiment 1
[0095] Such as figure 2 As shown, the improved DME edema area neural network segmentation model construction method provided by the embodiment of the present invention specifically includes:
[0096] 1.1 OCT image enhancement based on wavelet transform
[0097] OCT images have the characteristics of image noise and unclear lesion area, which affect the segmentation accuracy of DME edema area. Wavelet transform can denoise OCT images. Use wavelet transform to decompose the OCT image, and decompose the OCT image into a low-frequency sub-band and three high-frequency sub-bands, in which noise is mostly distributed in the low-frequency sub-band; edge and texture information are mostly distributed in the three high-frequency sub-bands; Through decomposition, the low-frequency sub-bands can be processed separately, and the noise in the low-frequency sub-bands can be removed without affecting the edge and texture information of the high-frequency sub-bands. If one-time decomposit...
Embodiment 2
[0123] 2.1 Accuracy evaluation
[0124] Using the dice coefficient DSC, precision Precision, and sensitivity Sensitive as the evaluation index of model segmentation performance, the calculation formula of each system is as follows:
[0125]
[0126]
[0127]
[0128] Among them, Vs and Vg represent the lesion area obtained by model segmentation and the lesion area obtained by visual interpretation, respectively.
PUM
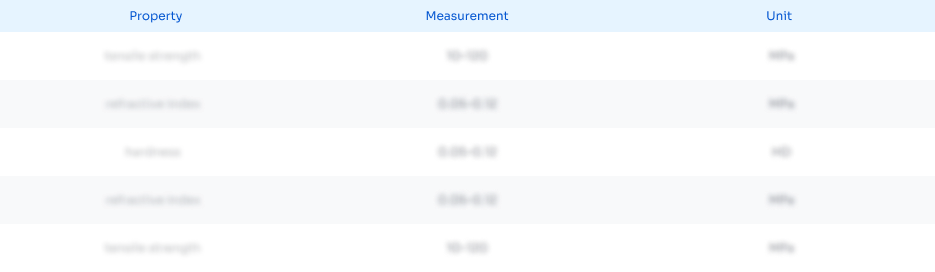
Abstract
Description
Claims
Application Information
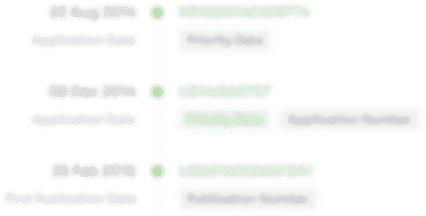
- R&D Engineer
- R&D Manager
- IP Professional
- Industry Leading Data Capabilities
- Powerful AI technology
- Patent DNA Extraction
Browse by: Latest US Patents, China's latest patents, Technical Efficacy Thesaurus, Application Domain, Technology Topic.
© 2024 PatSnap. All rights reserved.Legal|Privacy policy|Modern Slavery Act Transparency Statement|Sitemap