Improved YOLOv3 minimum remote sensing image target detection method and device and storage medium
A remote sensing image and target detection technology, applied in the field of target detection, can solve the problems of high false alarm rate, slow detection speed, and low detection rate of extremely small remote sensing image targets, and achieve the effect of improving detection speed and detection performance
- Summary
- Abstract
- Description
- Claims
- Application Information
AI Technical Summary
Problems solved by technology
Method used
Image
Examples
Example Embodiment
[0027] Example 1
[0028] This embodiment discloses an improved YOLOv3 method for detecting extremely small remote sensing image targets. The method includes the following steps:
[0029] S1 obtains the target data, and merges the convolutional layer feature output of the YOLOv3 network to form a pyramid feature layer;
[0030] S2 combines the feature output of the YOLOv3 shallow convolutional layer to form a pyramid feature layer;
[0031] S3 merges the bidirectional combination of the pyramid feature layer;
[0032] S4 changes the downsampling layer of the YOLOv3 network to a 3×3 convolutional layer;
[0033] S5 uses 1×1 convolution to reduce the dimension of the network model and output data.
[0034] In the S1, the convolutional layer feature output of the last layer of the YOLOv3 network is fused with the convolutional layer feature output of the adjacent upper layer to form a top-down pyramid feature layer.
[0035] In the S2, the convolutional layer feature output of the shallow lay...
Example Embodiment
[0038] Example 2
[0039] This embodiment discloses an improved YOLOv3 detection device for extremely small remote sensing image targets, including an FPN module, a memory, a processor, and a computer program stored in the memory and running on the processor. The computer program When executed by the processor, the improved YOLOv3 method for detecting targets in extremely small remote sensing images described in the foregoing embodiment is realized.
[0040] Add additional bottom-up, horizontal connection paths on the FPN module.
[0041] This embodiment also discloses a storage medium on which a computer program is stored, and when the computer program is executed by a processor, it implements the improved YOLOv3 method for detecting a very small remote sensing image target described in the above embodiment.
Example Embodiment
[0042] Example 3
[0043] In this embodiment, image classification can predict the category of a single target in the image, and target detection has its own challenges. It is necessary to predict multiple target categories and corresponding positions in a single image. In order to solve this problem, a pyramid feature method that represents the target feature layer at multiple scales is proposed. The feature pyramid network is one of the representative model architectures for generating the pyramid feature representation for target detection. It uses a top-down, horizontal connection and combination of two adjacent feature layers in the backbone network model to build a feature pyramid to generate high-resolution, strong semantic features. YOLOv3 draws on the idea of the above feature pyramid model, such as Figure 4 Shown. It is based on the backbone network model of Darknet53, with a total of 75 convolutional layers, 5 residual blocks, and 5 times of downsampling. It uses ...
PUM
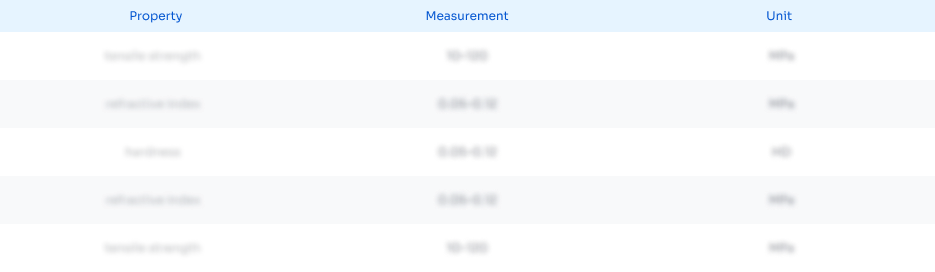
Abstract
Description
Claims
Application Information
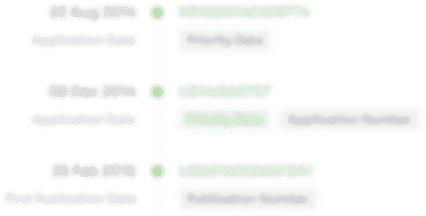
- R&D Engineer
- R&D Manager
- IP Professional
- Industry Leading Data Capabilities
- Powerful AI technology
- Patent DNA Extraction
Browse by: Latest US Patents, China's latest patents, Technical Efficacy Thesaurus, Application Domain, Technology Topic.
© 2024 PatSnap. All rights reserved.Legal|Privacy policy|Modern Slavery Act Transparency Statement|Sitemap