Optical orbital angular momentum machine learning recognition method based on turbulence effect
A technology of orbital angular momentum and machine learning, applied in the field of optical communication, can solve problems such as difficult learning, high training difficulty, and a large number of training samples, and achieve the effects of reducing learning difficulty, improving recognition accuracy, and shortening calculation time
- Summary
- Abstract
- Description
- Claims
- Application Information
AI Technical Summary
Problems solved by technology
Method used
Image
Examples
Embodiment 1
[0028] With the rapid development of optical communication technology, people's demand for information transmission volume and information security continues to increase, and the new degree of freedom of orbital angular momentum provides a new direction for people. Optical communication technology based on orbital angular momentum can greatly improve the information transmission capacity and spectrum utilization of communication, and has great development potential. Free space optical communication uses the atmosphere as a channel, but due to the complexity and randomness of atmospheric turbulence, it will cause mode crosstalk during orbital angular momentum transmission, thus increasing the bit error rate of optical communication systems based on orbital angular momentum. Therefore, the high-precision identification of orbital angular momentum under turbulent transmission of vortex beams is an important link to improve the link performance of optical communication systems. Th...
Embodiment 2
[0041] The optical orbital angular momentum machine learning identification method based on turbulence effect is the same as embodiment 1, adopts genetic algorithm in step 2 and utilizes feature vector to train more optimized support vector machine multi-classification model, specifically comprises the following steps:
[0042] (2a) Build a support vector machine multi-classification model: use the global construction method to construct a classifier to distinguish multi-class samples, and use Lagrangian expansion to obtain a support vector machine multi-classification model:
[0043]
[0044] In the formula, k represents the kth type, k=1,2,3,...,n; w k is the weight vector of the kth type; b k is the offset of the kth type; m=argmax{[(x·w 1 )+b 1 ],…,[(x·w n )+b n ]}, m≠k, x represents multi-class samples a i (i=1,2,3,...,l k ) represents the Lagrangian coefficient; C is the penalty factor; the kernel function K(x i ,x j ) Select the Radial basis kernel function...
Embodiment 3
[0050] The optical orbital angular momentum machine learning recognition method based on the turbulence effect is the same as that in embodiment 1-2, and the establishment of multiple models in step 4 performs group joint recognition of images with a larger recognition range, see Figure 5 , including the following steps:
[0051] (4a) Establish a joint recognition model: when the orbital angular momentum range of the light intensity distribution map to be recognized is large, set the recognition range to (1,2,...,a*b), referred to as the light intensity distribution map with a large recognition range Identify objects for a wide range. Divide the larger recognition range into b consecutive small ranges, namely (1,2,...,a), (a+1,a+2,...,2a),..., ((b-1 )*a+1,(b-1)*a+2,...a*b), referred to as the light intensity distribution map with a small recognition range is a small-range recognition object, where b is the number of small-range recognition objects, a Identify the number of ...
PUM
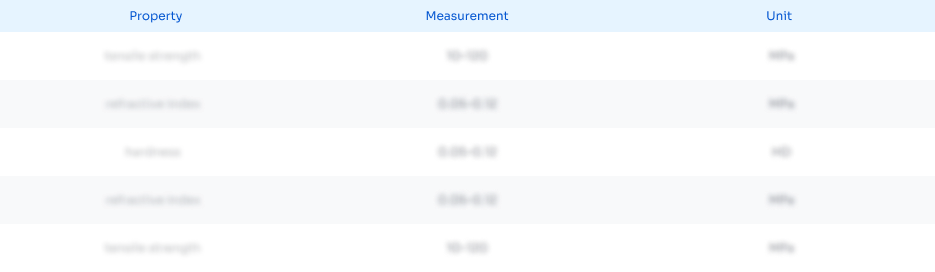
Abstract
Description
Claims
Application Information
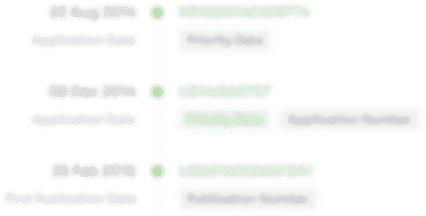
- R&D Engineer
- R&D Manager
- IP Professional
- Industry Leading Data Capabilities
- Powerful AI technology
- Patent DNA Extraction
Browse by: Latest US Patents, China's latest patents, Technical Efficacy Thesaurus, Application Domain, Technology Topic.
© 2024 PatSnap. All rights reserved.Legal|Privacy policy|Modern Slavery Act Transparency Statement|Sitemap