Blast furnace fault diagnosis rule exporting method based on deep neural network
A deep neural network and fault diagnosis technology, applied in neural learning methods, biological neural network models, computer systems based on knowledge-based models, etc. use, etc.
- Summary
- Abstract
- Description
- Claims
- Application Information
AI Technical Summary
Problems solved by technology
Method used
Image
Examples
Embodiment Construction
[0029] The purpose of the present invention is to provide a kind of blast furnace fault diagnosis rule derivation method based on deep neural network, the flow chart is as follows figure 1 As shown, considering the fragility and incompleteness of information in blast furnace systems, while taking advantage of the high diagnostic accuracy of deep neural networks, the abstract knowledge represented by deep neural network models is transformed into rules that are easily understood by blast furnace operators. It provides great convenience for blast furnace operators to understand, revise and learn from blast furnace fault diagnosis rules, and has strong practicability. The method can acquire knowledge from historical blast furnace fault data and transform it into a form that can be understood by operators, realize knowledge and decision enhancement in blast furnace fault diagnosis through man-machine collaboration, and ensure the reliability and accuracy of blast furnace fault diag...
PUM
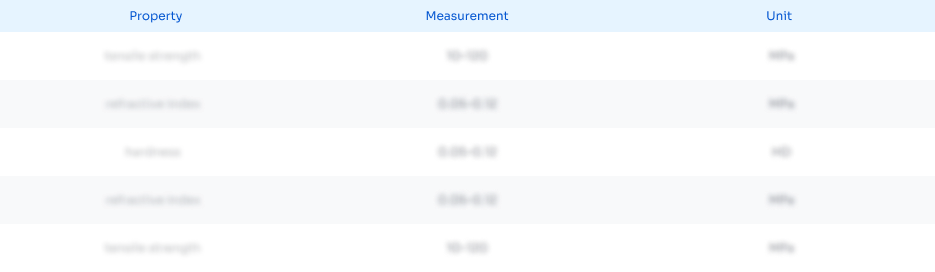
Abstract
Description
Claims
Application Information
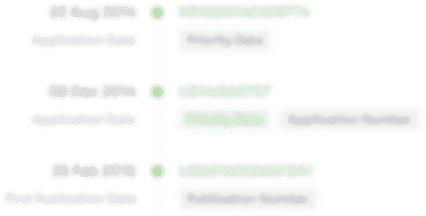
- R&D Engineer
- R&D Manager
- IP Professional
- Industry Leading Data Capabilities
- Powerful AI technology
- Patent DNA Extraction
Browse by: Latest US Patents, China's latest patents, Technical Efficacy Thesaurus, Application Domain, Technology Topic, Popular Technical Reports.
© 2024 PatSnap. All rights reserved.Legal|Privacy policy|Modern Slavery Act Transparency Statement|Sitemap|About US| Contact US: help@patsnap.com