Multi-class unbalanced remote sensing land cover image classification method based on integrated intervals
A technology of land cover and remote sensing images, which is applied in the field of multi-class imbalanced remote sensing classification, can solve the problems of small differences between base classifiers, heavy noise samples, and low operating efficiency, and achieve high classification accuracy, fast training speed, and Noise-capable effect
- Summary
- Abstract
- Description
- Claims
- Application Information
AI Technical Summary
Problems solved by technology
Method used
Image
Examples
Embodiment Construction
[0022] specific implementation plan
[0023] The embodiments and effects of the present invention will be described in detail below in conjunction with the accompanying drawings.
[0024] refer to figure 1 , the implementation steps of the present invention are as follows:
[0025] Step 1: Obtain unbalanced training samples.
[0026] Training samples are usually obtained from field surveys, manually extracted from high-spatial resolution images, or obtained from existing remote sensing image classification training databases. The training samples in this embodiment come from, but are not limited to, the Landsat satellite multispectral data of the UCI training database.
[0027] Step 2: Pre-classify the training samples using the random forest classification algorithm.
[0028] Commonly used classification algorithms for remote sensing images include maximum likelihood method, K-nearest neighbor method, and support vector machine.
[0029] This embodiment adopts but is not...
PUM
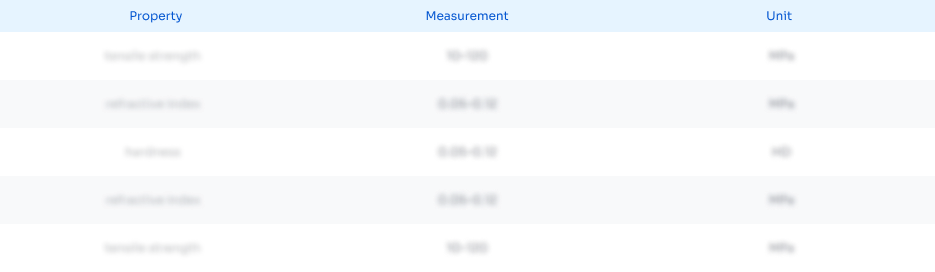
Abstract
Description
Claims
Application Information
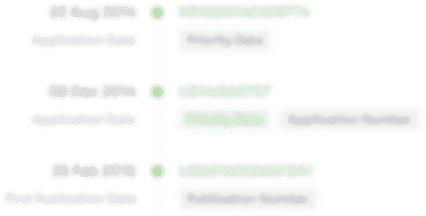
- R&D Engineer
- R&D Manager
- IP Professional
- Industry Leading Data Capabilities
- Powerful AI technology
- Patent DNA Extraction
Browse by: Latest US Patents, China's latest patents, Technical Efficacy Thesaurus, Application Domain, Technology Topic, Popular Technical Reports.
© 2024 PatSnap. All rights reserved.Legal|Privacy policy|Modern Slavery Act Transparency Statement|Sitemap|About US| Contact US: help@patsnap.com