Gas compressor vibration fault detection method based on recurrence plot and deep convolutional network
A technology of deep convolution and fault detection, applied in biological neural network models, computer components, neural learning methods, etc. Visually see the fault type and degree, etc., to achieve good fault detection effect, reduce huge workload, and avoid the effect of deviation
- Summary
- Abstract
- Description
- Claims
- Application Information
AI Technical Summary
Problems solved by technology
Method used
Image
Examples
Embodiment
[0044] The present invention will be further described below with specific examples. It should be noted that the following description is only used to explain the above-mentioned method of the present invention, rather than to limit the above-mentioned method of the present invention.
[0045] The specific embodiment of the present invention selects the aerodynamic instability data of a single-stage low-speed compressor test rig (Wang Cong, et al. Modeling and detection of rotational stall of axial flow compressor II: Experimental research based on Beihang low-speed compressor test rig. Control Theory and Applications, 2014.). The flow chart of vibration fault detection based on recurrent graph and deep convolutional neural network is shown in figure 1 shown, including the following steps:
[0046] Step 1. Create a database
[0047] The original data are 96 groups of timing signals (failure data in the process of instability development), and the duration of each group of te...
PUM
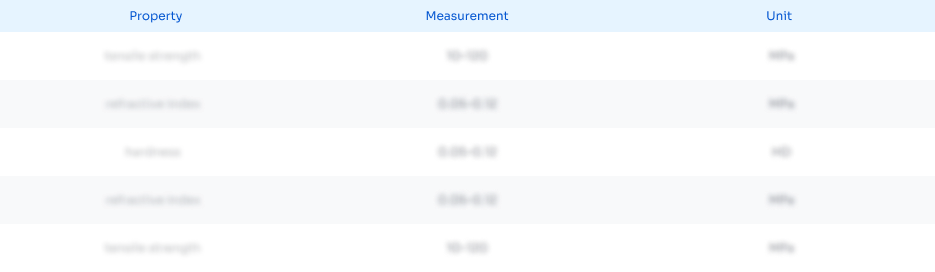
Abstract
Description
Claims
Application Information
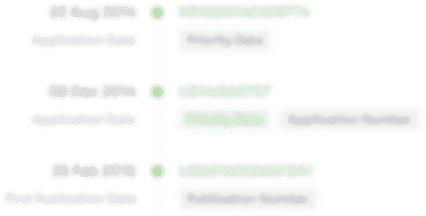
- R&D
- Intellectual Property
- Life Sciences
- Materials
- Tech Scout
- Unparalleled Data Quality
- Higher Quality Content
- 60% Fewer Hallucinations
Browse by: Latest US Patents, China's latest patents, Technical Efficacy Thesaurus, Application Domain, Technology Topic, Popular Technical Reports.
© 2025 PatSnap. All rights reserved.Legal|Privacy policy|Modern Slavery Act Transparency Statement|Sitemap|About US| Contact US: help@patsnap.com