End-to-end voice synthesis method and system based on DNN-HMM bimodal alignment network
A speech synthesis, dual-modal technology, applied in the field of intelligent speech interaction and computer intelligent speech synthesis, can solve the problems of unsatisfactory, unusable, and high model complexity of long sentence synthesis, to improve pronunciation, reduce complexity, The effect of reducing model parameters
- Summary
- Abstract
- Description
- Claims
- Application Information
AI Technical Summary
Problems solved by technology
Method used
Image
Examples
preparation example Construction
[0035] An end-to-end speech synthesis method based on a DNN-HMM bimodal alignment network mainly includes the following steps.
[0036]1. Convert the text into a phoneme input sequence, and convert the standard speech audio corresponding to the text into a standard mel spectrum.
[0037] 2. Text-to-speech alignment is performed through the DNN-HMM dual-modal alignment network to obtain a standard phoneme frame length sequence.
[0038] 3. Build a speech synthesis model.
[0039] 4. End-to-end training of the speech synthesis model.
[0040] 5. Convert the text to be processed into a phoneme input sequence to be processed and use it as the input of the trained speech synthesis model to obtain the corresponding speech of the text.
[0041] In a specific implementation of the present invention, the text preprocessing process is introduced.
[0042] Step 1-1, get the text data passed in by the interface, normalize the text, find out whether there are XML tags, if there are XML ...
Embodiment
[0125] In order to verify the implementation effect of the present invention, Figure 5 It is a test comparison made on the basis of domestic Chinese open source data. The Chinese open source data mainly uses the Chinese standard female voice database database open sourced by Biaobei Company. Its voice data is monophonic recording, using 48KHz 16-bit sampling frequency, PCM WAV format, a total of 10,000 sentences of Chinese girl voice data and corresponding text. Taking the open source data as the implementation method of this embodiment for further comparison and description, the specific data set segmentation method is shown in Table 1.
[0126] Table 1
[0127] data set training data Test Data Sampling Rate Biaobei open source female voice 9500 500 16K
[0128] According to the above-mentioned data distribution method, 9500 sentences are used as training data, and the training comparison is carried out with Tacotron2, the parameter method speec...
PUM
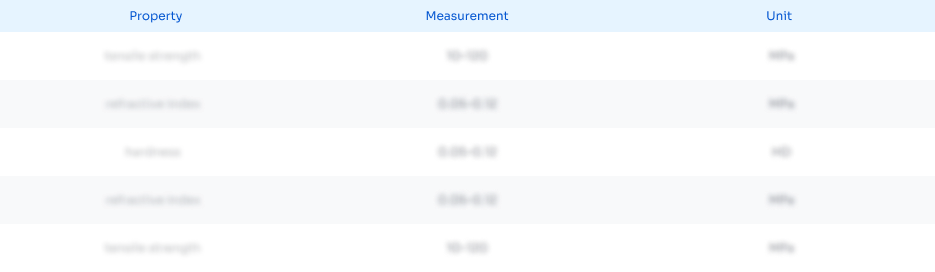
Abstract
Description
Claims
Application Information
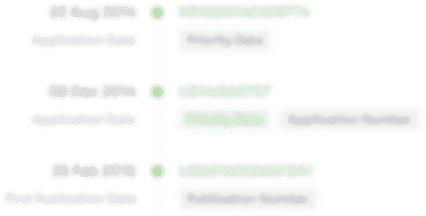
- R&D Engineer
- R&D Manager
- IP Professional
- Industry Leading Data Capabilities
- Powerful AI technology
- Patent DNA Extraction
Browse by: Latest US Patents, China's latest patents, Technical Efficacy Thesaurus, Application Domain, Technology Topic, Popular Technical Reports.
© 2024 PatSnap. All rights reserved.Legal|Privacy policy|Modern Slavery Act Transparency Statement|Sitemap|About US| Contact US: help@patsnap.com