Photovoltaic power station power generation sub-band prediction method based on improved EMD-LSTM combination model
A photovoltaic power station and prediction method technology, applied in the direction of prediction, neural learning methods, biological neural network models, etc., can solve the problems of complex environmental influence factors and poor accuracy, to solve the uncertain number of components, improve quality, and improve Effect of Time Series Prediction Effect
- Summary
- Abstract
- Description
- Claims
- Application Information
AI Technical Summary
Problems solved by technology
Method used
Image
Examples
Embodiment 1
[0045] Based on the improved EMD-LSTM combination model, the photovoltaic power generation frequency division prediction method, the photovoltaic power generation generation frequency division prediction method based on the improved EMD-LSTM combination model includes three stages:
[0046] First, neural network continuation and windowing are performed on the output data sequence of the photovoltaic power station to solve the problems of modal aliasing and pseudo-decomposition in the EMD decomposition, and the BP neural network is used to learn the terminal signal of the power station output sequence and reasonably predict the Sequence extreme points, extend the output curve to the left and right ends by several data points, so as to suppress the divergence of the end points. Further process the signal of the continuation part with a cosine window function, and multiply the continuation sequence with the cosine window function signal to reduce signal leakage. The combination o...
PUM
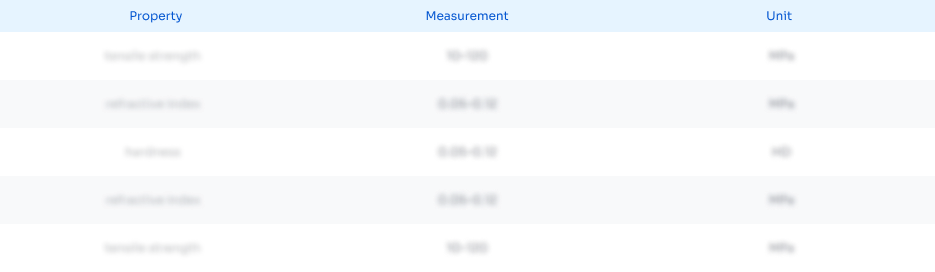
Abstract
Description
Claims
Application Information
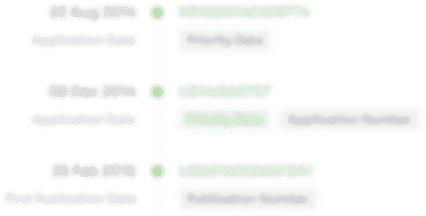
- R&D Engineer
- R&D Manager
- IP Professional
- Industry Leading Data Capabilities
- Powerful AI technology
- Patent DNA Extraction
Browse by: Latest US Patents, China's latest patents, Technical Efficacy Thesaurus, Application Domain, Technology Topic, Popular Technical Reports.
© 2024 PatSnap. All rights reserved.Legal|Privacy policy|Modern Slavery Act Transparency Statement|Sitemap|About US| Contact US: help@patsnap.com