Part machining surface prediction method based on space-time full convolutional recurrent neural network
A cyclic neural network and parts processing technology, applied in the field of image processing, can solve problems such as inability to share information between layers, meet the requirements of production takt, improve prediction accuracy and training speed, overcome the insufficiency of fitting ability and feature extraction ability Effect
- Summary
- Abstract
- Description
- Claims
- Application Information
AI Technical Summary
Problems solved by technology
Method used
Image
Examples
Embodiment 1
[0052] A method for predicting the machined surface of parts based on spatio-temporal fully convolutional cyclic neural network, said method comprising the following steps
[0053] (1) Generating grayscale images: By using three-dimensional high-resolution surface topography measurement technology to measure the top surface of the engine block, three-dimensional high-density point cloud data is obtained in sequence according to the processing sequence. The data is represented by X, Y, Z three-dimensional coordinates The format shows that the resolution in the X and Y directions is 150 μm, the measurement accuracy in the Z direction is 1 μm, the point cloud data density can reach 40 measurement points per square millimeter, and the total number of measurement points can reach 1 million points. The height value reflecting the surface topography of the part in the data is mapped to the gray value of the pixel to generate the gray image of the cylinder top surface during processing...
Embodiment 2
[0062] A part processing surface prediction method based on spatio-temporal full convolution cyclic neural network, said method comprises the following steps (1) acquiring a grayscale image: this embodiment is a grayscale image of a bearing side directly obtained by shooting with an industrial high-definition camera, Therefore, there is no need for 3D high-density point cloud data conversion.
[0063] (2) Obtain sub-atlas: take the image coordinates (668,300) as the initial sampling position, 64×64 pixels as the initial sampling size, and 32 pixels as the sampling interval to sample 10 times equidistantly, and obtain 10 sampling sub-images. The sampling results are as follows Figure 7 shown.
[0064] (3) Spatial autocorrelation analysis: analyze the spatial autocorrelation of 10 subgraphs. After the 10 sub-images were processed to remove the null value, their corresponding Moran indices were calculated, and the 256×256 pixels with the highest Moran index were taken as the op...
PUM
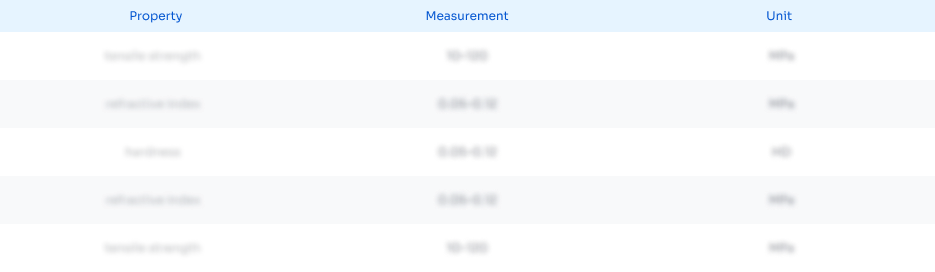
Abstract
Description
Claims
Application Information
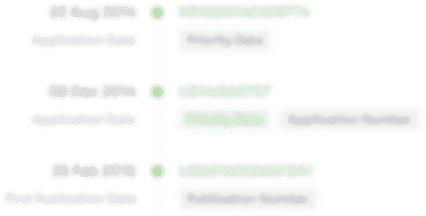
- Generate Ideas
- Intellectual Property
- Life Sciences
- Materials
- Tech Scout
- Unparalleled Data Quality
- Higher Quality Content
- 60% Fewer Hallucinations
Browse by: Latest US Patents, China's latest patents, Technical Efficacy Thesaurus, Application Domain, Technology Topic, Popular Technical Reports.
© 2025 PatSnap. All rights reserved.Legal|Privacy policy|Modern Slavery Act Transparency Statement|Sitemap|About US| Contact US: help@patsnap.com