Diagnostic device for detecting state of non-alcoholic fatty liver disease
A fatty liver disease, non-alcoholic technology, applied in measuring devices, medical automated diagnosis, biological testing, etc., can solve the problems of no accurate diagnostic methods available, and achieve fast and comprehensive evaluation and diagnosis, technical solutions and clear effects Effect
- Summary
- Abstract
- Description
- Claims
- Application Information
AI Technical Summary
Problems solved by technology
Method used
Image
Examples
Embodiment 1
[0084] Example 1 Research sample
[0085] The relevant experimental samples and methods of the present invention are as follows:
[0086] (1) Clinical samples
[0087] The clinical trial is a multi-center experiment, and the test samples in the present invention have been approved by the local ethics committee and the informed consent of all subjects has been obtained. Liver samples were obtained from adult patients who underwent liver biopsy and met clinical diagnostic criteria such as NAFLD, NASH; all subjects with the following conditions were excluded from the study: history of alcohol consumption, cancer, diabetes, or any other disease related to the liver. Peripheral venous blood samples were drawn after these subjects had fasted overnight for 12 hours.
[0088] According to the study protocol, the following analyzes were performed on all samples:
[0089] Hematology includes hemoglobin, hematocrit, RBC count, white blood cells, differential white blood cell count (ne...
Embodiment 2
[0102] Example 2 Establishment of Clinical Index Diagnosis Model
[0103] Use 70% of the training set data as the screening algorithm training, and 30% of the training set data as the algorithm test evaluation. In order to fully and reasonably compare the classification performance of the algorithm, the training data is randomly divided 100 times according to 7:3, and the decision tree (DT), random forest (RF), and gradient boosting tree (GBDT, here referred to as the GB model) are used to model 100 times. See the flow chart of prediction and algorithm training figure 1 .
[0104] Evaluation indicators and methods: You can choose the size of the area under the receiver operating characteristic curve (AUROC) as a judgment indicator to measure the classification performance of the classifier, and select the AUROC with the largest prediction and the largest average value of 100 predictions among the four algorithm models Design algorithms for optimal models.
[0105] The inve...
Embodiment 3
[0106] Example 3 Differentiate non-alcoholic simple fatty liver disease
[0107] Using a machine learning algorithm to establish a diagnostic model using clinical samples to evaluate hepatic steatosis, the gradient boosting tree model trained in the training set, preferably, can be analyzed by analyzing the subject's age, body mass index, fasting blood glucose, and gamma-glutamyl transfer Enzyme, high-density lipoprotein cholesterol, total bilirubin, albumin, aspartate aminotransferase, and platelet count nine clinical indicators, which output the possibility of subjects suffering from non-alcoholic simple fatty liver disease, That is to distinguish non-fatty liver (S0), mild fatty liver (S1), moderate fatty liver (S2) and severe fatty liver (S3), and find the best cut-off value through the Youden optimal point in ROC analysis, and diagnose no fatty liver. The cut-off value of fatty liver S0 and fatty liver patients is 2.63; the cut-off value of distinguishing non-fatty liver ...
PUM
Property | Measurement | Unit |
---|---|---|
Sensitivity | aaaaa | aaaaa |
Abstract
Description
Claims
Application Information
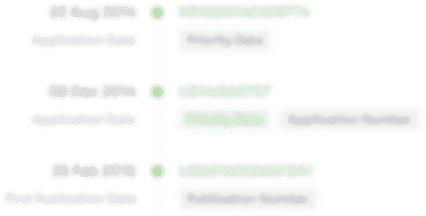
- R&D Engineer
- R&D Manager
- IP Professional
- Industry Leading Data Capabilities
- Powerful AI technology
- Patent DNA Extraction
Browse by: Latest US Patents, China's latest patents, Technical Efficacy Thesaurus, Application Domain, Technology Topic, Popular Technical Reports.
© 2024 PatSnap. All rights reserved.Legal|Privacy policy|Modern Slavery Act Transparency Statement|Sitemap|About US| Contact US: help@patsnap.com