Graph classification method based on attention mechanism and compound toxicity prediction method
A classification method and attention technology, applied in neural learning methods, computer components, biological neural network models, etc., can solve problems such as limited accuracy, model accuracy defects, and difficulty in processing nonlinear data.
- Summary
- Abstract
- Description
- Claims
- Application Information
AI Technical Summary
Problems solved by technology
Method used
Image
Examples
Embodiment Construction
[0048] Such as figure 1 Shown is a schematic flow chart of the classification method of the present invention: the attention mechanism-based graph classification method provided by the present invention includes the following steps:
[0049] S1. Obtain the original graph network and the corresponding graph network data information;
[0050] S2. According to the original graph network and data information obtained in step S1, initialize the feature embedding of nodes, construct a high-order graph network, and initialize the node embedding in the high-order graph network;
[0051] In the specific implementation, the following steps are used to initialize the feature embedding of the node:
[0052] For a given graph network G, let V(G) represent the node set of graph G, and E(G) represent the edge set of graph G; node i∈V(G) and edge (i,j)∈E(G) Both have attributes; encode the attributes of node i∈V(G) as u i N(i)={j|(i,j)∈E(G)} represents the neighbor nodes of node i, |N(i)| ...
PUM
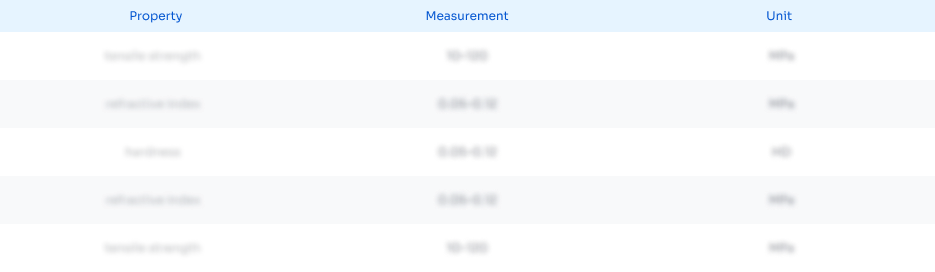
Abstract
Description
Claims
Application Information
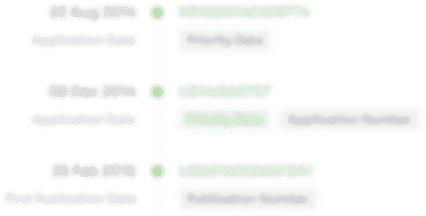
- R&D Engineer
- R&D Manager
- IP Professional
- Industry Leading Data Capabilities
- Powerful AI technology
- Patent DNA Extraction
Browse by: Latest US Patents, China's latest patents, Technical Efficacy Thesaurus, Application Domain, Technology Topic, Popular Technical Reports.
© 2024 PatSnap. All rights reserved.Legal|Privacy policy|Modern Slavery Act Transparency Statement|Sitemap|About US| Contact US: help@patsnap.com