A kind of remote sensing image cloud removal residual neural network system, method, device and storage medium based on multi-scale convolution and attention
A remote sensing image and neural network technology, applied in neural learning methods, biological neural network models, neural architectures, etc., can solve problems such as long computing time, large computing resource consumption, poor recovery effect, etc., to achieve strong learning ability and enhance adaptation. Effects of Sex and Robustness
- Summary
- Abstract
- Description
- Claims
- Application Information
AI Technical Summary
Problems solved by technology
Method used
Image
Examples
specific Embodiment approach 1
[0033] Embodiment 1: A high-resolution remote sensing image cloud removal residual neural network system based on multi-scale convolution and channel attention mechanism, the system includes an input module, a data enhancement module, a dimension enhancement module, a delicate feature module, a multi-dimensional A scale convolution module, a dimensionality reduction module and an output module; the above modules complete the logical link for a progressive relationship; the input module is responsible for collecting remote sensing image data; the data enhancement module is used to enhance the richness of the remote sensing image data enhancement operation obtained by the input module; The dimension increase module is responsible for increasing the dimension of the remote sensing image data; the delicate feature module is responsible for cropping the remote sensing image; the multi-scale convolution module is responsible for removing noise from the remote sensing image; the dimens...
specific Embodiment approach 2
[0034]Embodiment 2: In addition to the system flow of Embodiment 1, this embodiment proposes a method (MSAR_DefogNet) based on multi-scale convolution and attention to remove cloud residuals from remote sensing images. The richer dataset PRSC for the image de-cloud task. The multi-scale convolution block in the MSAR_DefogNet model can extract features of different scales and fuse these features to achieve efficient cloud removal. The residual block with channel attention mechanism can extract detailed features and realize the function of mining detailed information. Using the PRSC dataset can enhance the adaptability and robustness of the model, which helps to transfer the model to real-world scenarios. Compared with the existing algorithms, this method does not require explicit estimation of the transmission rate, etc., has a stronger learning ability, can adapt to a variety of scene changes, has better image restoration effect and faster processing speed, and has a high imp...
specific Embodiment approach 3
[0073] Specific embodiment three: the above method examples can be attached according to the description figure 1 The block diagram shown is for the division of functional modules. For example, each functional module can be divided according to each function, or two or more functions can be integrated into one processing module; the above-mentioned integrated modules can be implemented in the form of hardware, It can also be implemented in the form of software function modules. It should be noted that, the division of modules in the embodiment of the present invention is schematic, and is only a logical function division, and there may be other division manners in actual implementation.
[0074] Specifically, the system is carried on a computer, including a processor, a memory, a bus and a communication device;
[0075] The memory is used to store computer-executed instructions, the processor is connected to the memory through the bus, the processor executes the computer-exec...
PUM
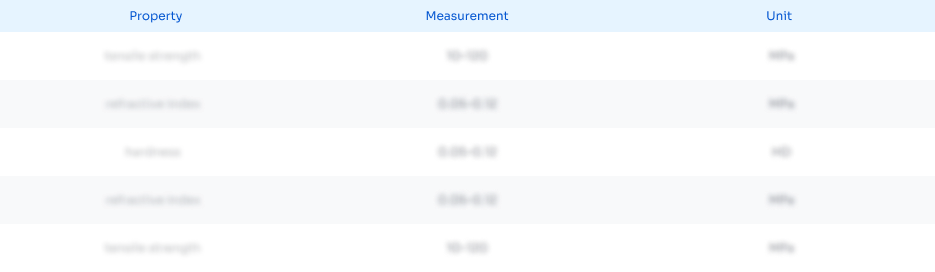
Abstract
Description
Claims
Application Information
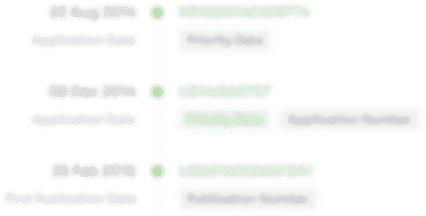
- R&D Engineer
- R&D Manager
- IP Professional
- Industry Leading Data Capabilities
- Powerful AI technology
- Patent DNA Extraction
Browse by: Latest US Patents, China's latest patents, Technical Efficacy Thesaurus, Application Domain, Technology Topic, Popular Technical Reports.
© 2024 PatSnap. All rights reserved.Legal|Privacy policy|Modern Slavery Act Transparency Statement|Sitemap|About US| Contact US: help@patsnap.com