Driver model optimization method based on GRU
A technology of driver model and optimization method, applied in the field of driver model optimization based on GRU, can solve problems such as deviation and inability to apply driver model, and achieve the effect of reducing deviation, reducing traffic accidents and avoiding fatigue
- Summary
- Abstract
- Description
- Claims
- Application Information
AI Technical Summary
Problems solved by technology
Method used
Image
Examples
Embodiment 1
[0031] figure 1 and figure 2 A GRU-based driver model optimization method is provided, which uses pre-set road trajectory and expected vehicle speed information to establish a driver's preview following model, uses the model to determine the expected value of the driver's steering wheel angle applied to the car, and uses The expected path of the driver is obtained by fitting the two-degree-of-freedom vehicle model. Using the Gated Recurrent Unit (GRU) network, the desired path decision-making process is trained with a multi-factor weight-adjusted deep neural network, thereby reducing the deviation between the expected path and the actual path, and obtaining a good preview path following driving member model. The present invention can be applied to the field of smart cars in practical applications, and provides technical solutions for some technical problems in the fields of automatic driving, driving assistance, and airline supervision. The optimal path with high matching d...
Embodiment 2
[0143] The general steps of this embodiment adopt the content described in Embodiment 1, wherein the values of the vehicle parameters used for simulation and the actual path parameters are as follows, and the driver model based on GRU optimization is obtained through the method described in Embodiment 1 The preview path of the decision, here the optimized preview path is called the comparison result of the GRU path and the actual path simulated by the driving simulator. However, it should be noted that the vehicle parameters and the actual path of the simulation can be changed arbitrarily, and this embodiment only shows the simulation experiment results under one set of parameters.
[0144] Vehicle parameter determination:
[0145] Vehicle mass m = 1301kg;
[0146] Wheelbase L=2.537m;
[0147] Front wheelbase a=1.074m;
[0148] Rear wheelbase b=1.463m;
[0149] Vehicle yaw moment of inertia I Z =1600kg·m 2 ;
[0150] Front and rear axle cornering stiffness k 1 ,k 2 ...
Embodiment 3
[0162] A GRU-based driver model optimization system is provided, which includes an expected path generation module, a driver model generation module, a two-degree-of-freedom vehicle model generation module, a GRU module and an output module.
[0163] The expected path generation module generates the actual path through simulation, or can also retrieve the actual collected path data in the training library, and transmit the expected path to the driver model generation module.
[0164] The preset program in the driver model generation module establishes the driver model based on the preview follow according to the actual path, and analyzes the lateral displacement process of the vehicle and corrects the analysis results according to the driver's hysteresis effect transfer function and lateral acceleration error feedback. Get the steering wheel angle as δ sw And output to the two-degree-of-freedom vehicle model generation module.
[0165] The two-degree-of-freedom vehicle model ...
PUM
Property | Measurement | Unit |
---|---|---|
Wheelbase | aaaaa | aaaaa |
Abstract
Description
Claims
Application Information
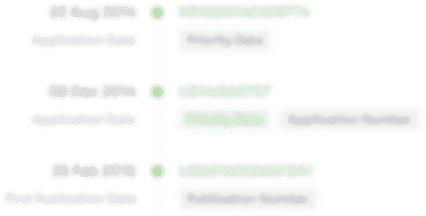
- R&D
- Intellectual Property
- Life Sciences
- Materials
- Tech Scout
- Unparalleled Data Quality
- Higher Quality Content
- 60% Fewer Hallucinations
Browse by: Latest US Patents, China's latest patents, Technical Efficacy Thesaurus, Application Domain, Technology Topic, Popular Technical Reports.
© 2025 PatSnap. All rights reserved.Legal|Privacy policy|Modern Slavery Act Transparency Statement|Sitemap|About US| Contact US: help@patsnap.com