Cancer classification and characteristic gene selection method
A technology for eigengenes and cancer, applied in the field of biological information, can solve problems such as unstable prediction performance, and achieve the effect of enhancing interpretability, improving accuracy and stability, and improving model accuracy
- Summary
- Abstract
- Description
- Claims
- Application Information
AI Technical Summary
Problems solved by technology
Method used
Image
Examples
Embodiment Construction
[0054] The present invention is described in detail below with reference to accompanying drawing and embodiment:
[0055] attached figure 1 and 2 It can be seen that a cancer classification and feature gene selection method includes the following steps:
[0056] (1) The establishment of the primary learner:
[0057] For n*p-dimensional training set matrix X and sample label y, establish T logistic regression models as primary learners;
[0058] For the sparse group lasso (SGL) regularization term, it has a mixed parameter α to adjust the weight of lasso and group lasso, based on T equidistant distribution between (0, 1) α value, establish corresponding T SGL Regularized logistic regression solution model;
[0059] for each α t , choose the optimal regularization parameter λ by cross-validation t , and record the predicted probability value of the validation set in each primary learner as n*T matrix
[0060] (2) The establishment of the secondary learner:
[0061] Est...
PUM
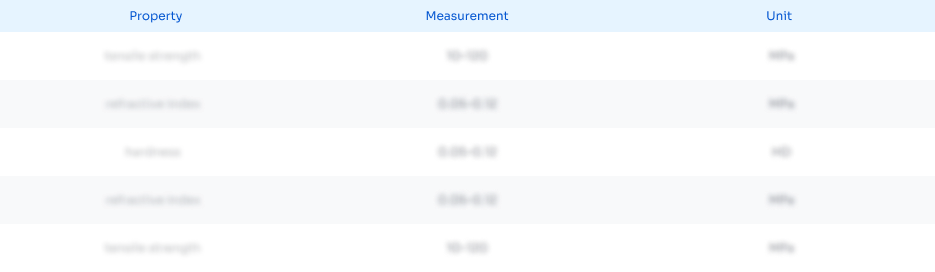
Abstract
Description
Claims
Application Information
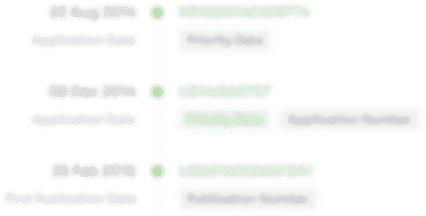
- R&D
- Intellectual Property
- Life Sciences
- Materials
- Tech Scout
- Unparalleled Data Quality
- Higher Quality Content
- 60% Fewer Hallucinations
Browse by: Latest US Patents, China's latest patents, Technical Efficacy Thesaurus, Application Domain, Technology Topic, Popular Technical Reports.
© 2025 PatSnap. All rights reserved.Legal|Privacy policy|Modern Slavery Act Transparency Statement|Sitemap|About US| Contact US: help@patsnap.com