Disease classification method fusing multi-modal features based on integrated learning and equipment
An integrated learning and disease classification technology, applied in character and pattern recognition, instruments, computer parts, etc., can solve the problems of insufficient use of medical imaging data mining, only considering single-modal image features, and insufficient classification accuracy. Good self-learning ability, improve classification accuracy and model generalization ability, and avoid the effect of prediction errors
- Summary
- Abstract
- Description
- Claims
- Application Information
AI Technical Summary
Problems solved by technology
Method used
Image
Examples
Embodiment 1
[0037] This embodiment provides a disease classification method based on integrated learning and fusion of multi-modal features. This embodiment applies it to the classification of early Parkinson's disease (Parkinson's Disease, PD), including the following steps:
[0038] Step 1: Acquire multimodal magnetic resonance images and clinical text information of the same subject obtained on the same device, and perform data preprocessing.
[0039] Multimodal magnetic resonance images include T1-weighted magnetic resonance imaging (T1-WI), diffusion tensor imaging (DTI) and resting functional magnetic resonance imaging (resting functional magnetic resonance imaging, rs-fMRI) three modality image data. The clinical text information selects pre-clinical non-motor disorder score data. Corresponding image preprocessing is performed on the three modal magnetic resonance imaging data, and data normalization preprocessing is performed on the clinical text information.
[0040] (1) T1-WI ...
Embodiment 2
[0060] This embodiment also provides a disease classification device based on integrated learning and fusion of multi-modal features, including data screening and preprocessing modules, feature extraction modules, adaptive base classifier selection modules and meta-learner integrated learning modules, wherein data screening And the preprocessing module is used to obtain T1-weighted magnetic resonance images, diffusion tensor images, resting state functional magnetic resonance images and pre-clinical non-movement scores, and perform corresponding image preprocessing on the three modal magnetic resonance imaging data, Perform data normalization preprocessing on clinical text information; the feature extraction module is used to extract the whole brain morphological feature map of each modality image, and calculate the morphological feature values in the brain area of interest, and use the double-sample t-test statistics The clinical text information with inter-group differenc...
Embodiment 3
[0062] The present invention also provides an electronic device, including one or more processors, memory, and one or more programs stored in the memory, one or more programs include a method for performing the integrated learning based on the method described in Embodiment 1. Instructions for Disease Classification Methods Fused with Multimodal Features.
PUM
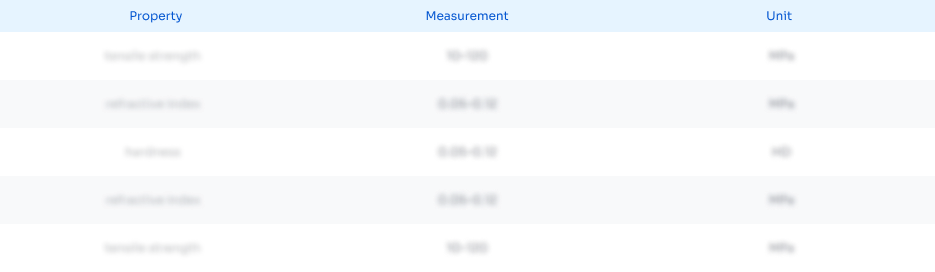
Abstract
Description
Claims
Application Information
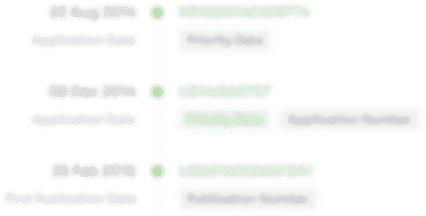
- R&D Engineer
- R&D Manager
- IP Professional
- Industry Leading Data Capabilities
- Powerful AI technology
- Patent DNA Extraction
Browse by: Latest US Patents, China's latest patents, Technical Efficacy Thesaurus, Application Domain, Technology Topic, Popular Technical Reports.
© 2024 PatSnap. All rights reserved.Legal|Privacy policy|Modern Slavery Act Transparency Statement|Sitemap|About US| Contact US: help@patsnap.com