Image semantic segmentation method based on multi-receptive-field context semantic information
A semantic information, multi-receptive field technology, applied in the field of image recognition, can solve the problems of large memory, consumption, etc., to achieve the advantages of accuracy, low memory consumption, and convenient implementation.
- Summary
- Abstract
- Description
- Claims
- Application Information
AI Technical Summary
Problems solved by technology
Method used
Image
Examples
Embodiment Construction
[0035] Such as figure 1 As shown, the image semantic segmentation method based on multi-receptive field context semantic information of the present invention comprises the following steps:
[0036] Step 1. Convert the input image into a pixel matrix through a convolution operation;
[0037] Step 2. Using dilated convolutions with different dilation rates to convert the same pixel matrix into multiple feature maps with contextual semantic information of multiple receptive fields;
[0038] The output of the dilated convolution is:
[0039]
[0040] Among them, y i Represents the i-th output of the expanded convolution, the convolution kernel size of the expanded convolution is k*k, and the expansion rate is r,x i is the i-th input feature map of the dilated convolution before the converter subnetwork, and m is the length of the filter matrix w[k] with a convolution kernel size of k*k.
[0041] The receptive field is very important in target detection and image segmentation....
PUM
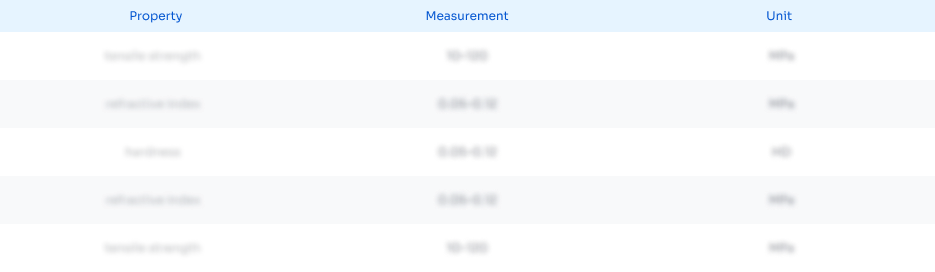
Abstract
Description
Claims
Application Information
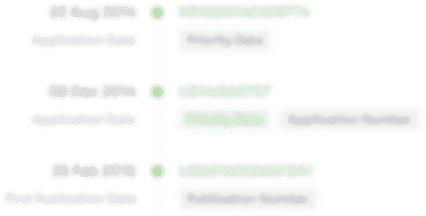
- R&D Engineer
- R&D Manager
- IP Professional
- Industry Leading Data Capabilities
- Powerful AI technology
- Patent DNA Extraction
Browse by: Latest US Patents, China's latest patents, Technical Efficacy Thesaurus, Application Domain, Technology Topic.
© 2024 PatSnap. All rights reserved.Legal|Privacy policy|Modern Slavery Act Transparency Statement|Sitemap