Deep learning prediction method of target-ligand binding affinity based on gated attention mechanism
A technology of deep learning and prediction methods, applied in neural learning methods, used to analyze two-dimensional or three-dimensional molecular structures, informatics, etc., can solve the problem that large data sets are difficult to balance accuracy and versatility, and 3D structural features are not easy to obtain and other problems to achieve the effect of saving computing time and cost and high interpretability
- Summary
- Abstract
- Description
- Claims
- Application Information
AI Technical Summary
Problems solved by technology
Method used
Image
Examples
Embodiment
[0068] The target-ligand complexes were randomly divided into training, validation, and test sets in a ratio of 8:1:1. The number of samples in the training, validation, and test sets are 6,711, 841, and 841, respectively. There are 3,102 low binding affinity complexes (label "0") and 3,609 high binding affinity complexes (label "1") in the training set, meeting the data balance requirement for deep learning classification models. The loss function in our deep learning model is the cross entropy loss (CEL), which is a commonly used loss function in classification tasks. To minimize the loss function, the model parameters are optimized by using the Adaptive Moment Estimation (Adam) optimizer with a learning rate setting varying from 0.0005 to 0.00005, where a decay factor (0.5) is used when the loss function on the validation set does not decrease with iterations to update the learning rate. Generally speaking, 50% is the threshold for judging true and false in a binary class...
PUM
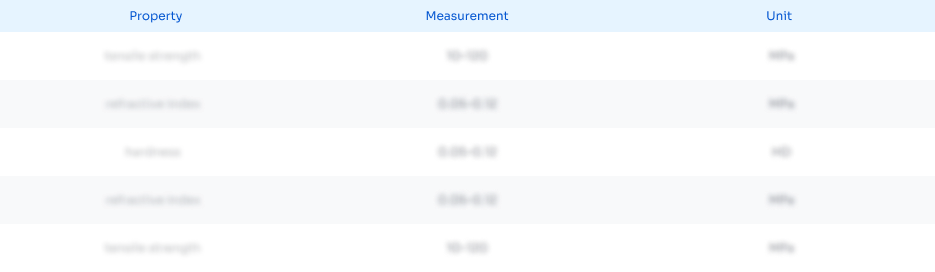
Abstract
Description
Claims
Application Information
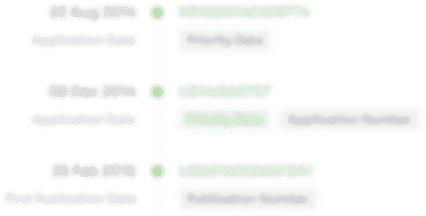
- R&D Engineer
- R&D Manager
- IP Professional
- Industry Leading Data Capabilities
- Powerful AI technology
- Patent DNA Extraction
Browse by: Latest US Patents, China's latest patents, Technical Efficacy Thesaurus, Application Domain, Technology Topic, Popular Technical Reports.
© 2024 PatSnap. All rights reserved.Legal|Privacy policy|Modern Slavery Act Transparency Statement|Sitemap|About US| Contact US: help@patsnap.com