Gene selection method based on feature discrimination and independence
A gene selection and identification technology, applied in special data processing applications, instruments, electrical digital data processing, etc., can solve problems such as overfitting of small sample data sets and high time overhead.
- Summary
- Abstract
- Description
- Claims
- Application Information
AI Technical Summary
Problems solved by technology
Method used
Image
Examples
Embodiment 1
[0067] In this embodiment, the feature selection method based on feature recognition and independence is implemented by the following steps:
[0068] (1) Randomly generate the first type of data set D conforming to the normal distribution 1 , denoted as D 1 ={X 1 ;X 2 ;…;X 10}∈R 10 ×50 , randomly generate the second type of data set D conforming to the normal distribution 2 , denoted as D 2 ={X 11 ;X 12 ;…;X 20}∈R 10×50 , data set D 1 and D 2 Each contains 10 samples, and each sample has 50 features. Dataset D 1 and D 2 Merge into a data set D, expressed as D={X 1 ;X 2 ;…;X 20}∈R 20×50 , which contains 20 samples, distributed in 2 categories, each sample contains 50 features, and then use the bootstrap method to divide the data set to obtain the training set and test set.
[0069] (2) Calculate the recognition degree of each feature
[0070] (2.1) Use the Wilcoxon rank sum test method to calculate the weight w of each feature in the data set D i ,specific...
Embodiment 2
[0089] In step (2) of this embodiment, the weight w of each feature in the data set D i The calculation method of can also be calculated by the D-Score method. D-Score is a feature weight calculation method based on intra-class and inter-class distances. The specific calculation formula is as follows:
[0090]
[0091] Among them, D i Indicates that the fth in the data set D i The D-Score value of a feature, that is, the fth i The weight of features, c is the number of categories in the data set, are the mean values of the i-th feature on the entire data set and the j'th class data set, respectively, is the eigenvalue of the i-th feature of the v-th sample point in the j'th class.
[0092] Other steps are identical with embodiment 1.
Embodiment 3
[0094] In step (2) of this embodiment, the weight w of each feature in the data set D i The calculation method of can also be calculated by the method based on mutual information. Mutual information is used to evaluate the correlation between two features or between features and class labels. The calculation formula is as follows:
[0095] I(f i ,Y)=H(Y)-H(Y|f i )
[0096] Among them, Y represents the class label vector of the data set; I(f i , Y) represents the feature f in the data set i The mutual information value between and the class label vector Y, that is, the feature f i weight; H(Y) is the information entropy of the class label vector Y; H(Y|f i ) for the feature f i The information entropy of the class label vector Y under the condition of determining the value.
[0097] For continuous features, it needs to be discretized in advance.
[0098] Other steps are identical with embodiment 1.
PUM
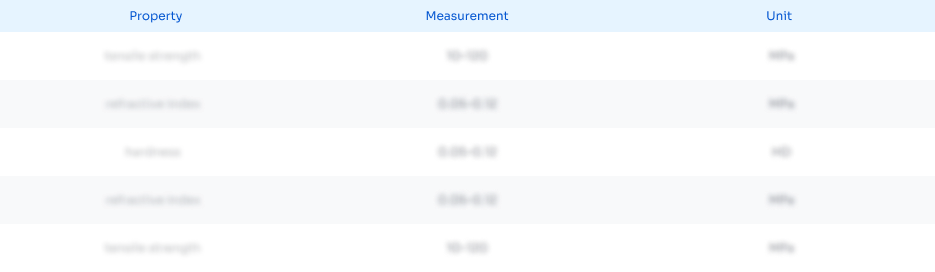
Abstract
Description
Claims
Application Information
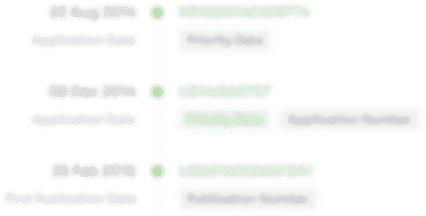
- R&D Engineer
- R&D Manager
- IP Professional
- Industry Leading Data Capabilities
- Powerful AI technology
- Patent DNA Extraction
Browse by: Latest US Patents, China's latest patents, Technical Efficacy Thesaurus, Application Domain, Technology Topic, Popular Technical Reports.
© 2024 PatSnap. All rights reserved.Legal|Privacy policy|Modern Slavery Act Transparency Statement|Sitemap|About US| Contact US: help@patsnap.com