Markov chain Monte Carlo automatic history fitting method and system based on t distribution
A Markov chain and history fitting technology, applied in special data processing applications, instruments, electrical digital data processing, etc., can solve problems such as strong uncertainty, large amount of simulated annealing calculations, and unsatisfactory Gaussian distribution, etc., to achieve Effects of improving efficiency and accuracy and shortening fitting time
- Summary
- Abstract
- Description
- Claims
- Application Information
AI Technical Summary
Problems solved by technology
Method used
Image
Examples
Embodiment 1
[0081] Embodiment 1. Markov chain Monte Carlo automatic history fitting method based on t distribution. Combine below Figure 1 to Figure 16 The method provided in this embodiment will be described in detail.
[0082] see Figure 1 to Figure 3 , S1. The initial static parameters of the reservoir are obtained by random initialization with t distribution.
[0083] Specifically, the parameters that need to be optimized in the reservoir numerical model are the static parameters of the reservoir, such as permeability and porosity, etc., which are divided into grids. The initial values are randomly assigned through a certain probability distribution model. Traditional methods often use Gaussian distribution to obtain the permeability. However, due to the strong heterogeneity of the reservoir, especially after repeated oil recovery after repeated water injection and chemical agent injection, the uncertainty of various physical properties in the reservoir is strong, and the charac...
specific example
[0154] 1. To test the effect of the automatic history fitting method of Markov chain Monte Carlo reservoir parameters based on t-distribution. The PUNQ-S3 reservoir data model was used in the experiment. The PUNQ-S3 reservoir data model is a three-dimensional three-phase reservoir engineering model, which consists of 19*28*25 grid blocks and is divided into five layers. Each layer There are 2660 grid blocks, and each grid block has the same size, which contains 1761 valid grid blocks. Such as figure 2 As shown, the blank part represents the invalid grid, and the grid with line segment characteristics represents the horizontal permeability of different values. For each layer of the model, the horizontal permeability can be divided into different blocks. In summary, The 1761 grids of the PUNQS3 reservoir model can be divided into 45 blocks of 5*9 to achieve the purpose of history matching partition and block. The horizontal permeability distribution of each layer in the PUNQS...
Embodiment 2
[0177] Embodiment 2. Markov chain Monte Carlo automatic history fitting system based on t distribution. Combine below Figure 17 to Figure 20 The system provided in this embodiment will be described in detail.
[0178] see Figure 17 to Figure 20 , a Markov chain Monte Carlo automatic history fitting system based on t distribution, characterized in that the system includes an initialization module, a construction module, an optimization module, a posteriori estimation module and an output module.
[0179] The initialization module is used to obtain the initial static parameters of the reservoir by random initialization with t distribution.
[0180] The construction module is used to construct the objective function of the reservoir model according to the Bayesian formula.
[0181] Specifically, the construction module specifically includes a first construction unit, a second construction unit, and an objective function construction unit.
[0182] The first structural unit ...
PUM
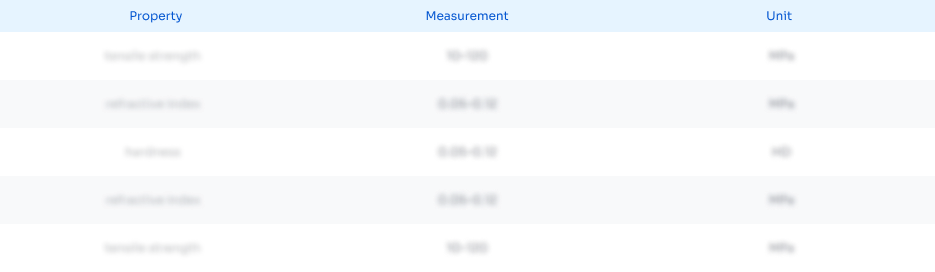
Abstract
Description
Claims
Application Information
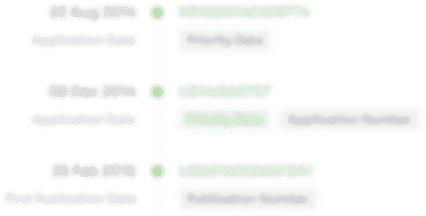
- R&D
- Intellectual Property
- Life Sciences
- Materials
- Tech Scout
- Unparalleled Data Quality
- Higher Quality Content
- 60% Fewer Hallucinations
Browse by: Latest US Patents, China's latest patents, Technical Efficacy Thesaurus, Application Domain, Technology Topic, Popular Technical Reports.
© 2025 PatSnap. All rights reserved.Legal|Privacy policy|Modern Slavery Act Transparency Statement|Sitemap|About US| Contact US: help@patsnap.com