Local and non-local multi-feature semantics-based hyperspectral image classification method
A technology of hyperspectral images and classification methods, applied in the field of hyperspectral image classification based on local and non-local multi-feature semantics, can solve the problems of poor robustness, low classification result accuracy, weak spatial consistency of classification result graphs, etc. Robustness, avoid falling into local optimal solution, improve the effect of spatial consistency
- Summary
- Abstract
- Description
- Claims
- Application Information
AI Technical Summary
Problems solved by technology
Method used
Image
Examples
Embodiment 1
[0028] For the classification of ground objects in hyperspectral images, most of the current existing methods have the problems of unsatisfactory classification accuracy, poor robustness of classification results, and weak spatial consistency of classification result maps. The present invention combines various features in The fusion technology of semantic space and the local and non-local space constraint method mainly propose a semantic hyperspectral image classification method based on local and non-local multi-features to solve various problems existing in the existing methods.
[0029] The present invention is a semantic hyperspectral image classification method based on local and non-local multi-features, see figure 1 , including the following steps:
[0030] (1) Input an image and extract various features of the image.
[0031] Commonly used hyperspectral image data include the Indian Pine dataset and the Salinas dataset obtained by the airborne visible / infrared imagin...
Embodiment 2
[0046] Based on local and non-local multi-feature semantic hyperspectral image classification method with embodiment 1, wherein the multiple features described in step (1), including but not limited to: original spectral features, Gabor texture features, differential morphology features (DMP ), where Gabor texture features and differential morphology features (DMP) are expressed as follows:
[0047] Gabor texture features: for hyperspectral images Perform principal component analysis (PCA) processing, take the processed first 3-dimensional principal components as 3 reference images, and perform Gabor transformation in 16 directions and 5 scales respectively, and obtain 80-dimensional texture features for each reference image, and stack Gabor texture features with a total dimension of 240 dimensions are obtained together.
[0048] Differential Morphological Features (DMP): For hyperspectral images Perform principal component analysis (PCA) processing, take the processed fir...
Embodiment 3
[0052] Based on local and non-local multi-feature semantic hyperspectral image classification method with embodiment 1-2, wherein the local and non-local neighbor set construction method described in step (4) is as follows:
[0053] 4a) For the hyperspectral image Perform principal component analysis (PCA), extract the first principal component as a reference image, that is, a grayscale image that can reflect the basic contour information of the hyperspectral image, set the number of superpixels LP, and perform entropy-based The superpixel image segmentation of , get LP superpixel blocks
[0054] In this embodiment, the entropy-based superpixel segmentation method is used to perform superpixel segmentation on the first principal component grayscale image of the hyperspectral image, and the segmented superpixel blocks can well maintain the edge information and structural information in the image, and the segmentation The size difference of the superpixel block is small, and...
PUM
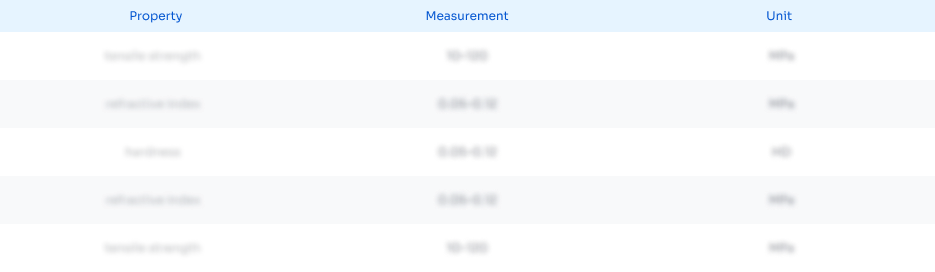
Abstract
Description
Claims
Application Information
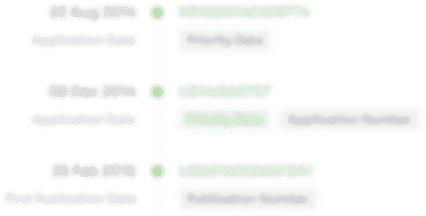
- R&D Engineer
- R&D Manager
- IP Professional
- Industry Leading Data Capabilities
- Powerful AI technology
- Patent DNA Extraction
Browse by: Latest US Patents, China's latest patents, Technical Efficacy Thesaurus, Application Domain, Technology Topic.
© 2024 PatSnap. All rights reserved.Legal|Privacy policy|Modern Slavery Act Transparency Statement|Sitemap