Granular support vector machine ensemble-based protein ligand binding site prediction method
A technology of support vector machines and protein ligands, which is applied in the field of protein ligand binding site prediction based on granular support vector machine integration, can solve problems such as class imbalance and information redundancy in data sets, and achieve enhanced generalization ability. , Improve the prediction accuracy and prevent the effect of overfitting
- Summary
- Abstract
- Description
- Claims
- Application Information
AI Technical Summary
Problems solved by technology
Method used
Image
Examples
Embodiment Construction
[0022]The present invention will be further described below in conjunction with the accompanying drawings and specific embodiments.
[0023] Such as figure 1 As shown, according to a preferred embodiment of the present invention, the protein ligand binding site prediction method based on granular support vector machine integration is used for a protein sequence to be predicted / queried (hereinafter referred to as a given query input q) Prediction, which is divided into five steps, the first four steps are the model training stage, and the fifth step is the prediction stage, combined below figure 1 As shown, the implementation of the above five steps is described in detail.
[0024] The first step is to perform feature extraction based on the evolutionary information and secondary structure of the existing protein sequence, express the amino acid residues in the sequence in the form of feature vectors, and construct a training sample set in units of residues (sites). For any g...
PUM
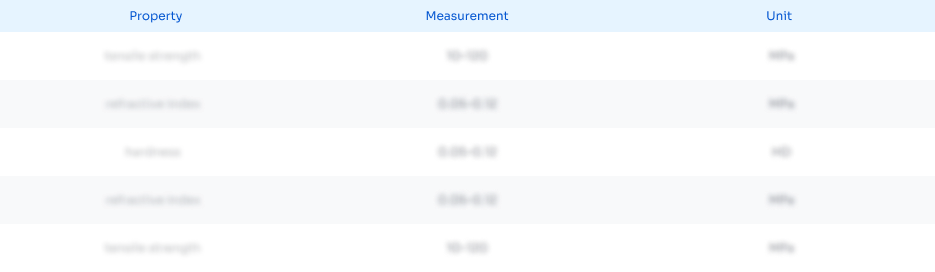
Abstract
Description
Claims
Application Information
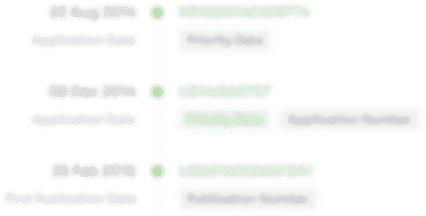
- R&D Engineer
- R&D Manager
- IP Professional
- Industry Leading Data Capabilities
- Powerful AI technology
- Patent DNA Extraction
Browse by: Latest US Patents, China's latest patents, Technical Efficacy Thesaurus, Application Domain, Technology Topic, Popular Technical Reports.
© 2024 PatSnap. All rights reserved.Legal|Privacy policy|Modern Slavery Act Transparency Statement|Sitemap|About US| Contact US: help@patsnap.com