Acoustic model modeling method of residual error long short-term memory recurrent neural network
A technology of cyclic neural network and long-term and short-term memory, applied in speech analysis, speech recognition, instruments, etc., can solve the problems of gradient explosion, gradient disappearance, acoustic environment affecting the recognition accuracy of speech recognition system, etc., to improve performance and prevent over-simulation The effect of solving the problem and improving the generalization ability of the model
- Summary
- Abstract
- Description
- Claims
- Application Information
AI Technical Summary
Problems solved by technology
Method used
Image
Examples
Embodiment Construction
[0034] The implementation of the present invention will be described in detail below in conjunction with the drawings and examples.
[0035] The present invention proposes a method and device for a residual long-short-term memory cyclic neural network acoustic model, especially for continuous speech recognition scenarios. These methods and devices are not limited to continuous speech recognition, and may be any methods and devices related to speech recognition.
[0036] figure 1 It is a flow chart of the acoustic model of the residual long short-term memory recurrent neural network of the present invention, including the following:
[0037] Such as figure 1 The input 101 shown is the speech signal feature x t ; Others are residual long short-term memory cyclic neural network submodule 102, which is composed of memory cell 103, input gate 104, output gate 105, forgetting gate 106, multiplier 107; the output of long short-term memory neural network submodule 102 As the input...
PUM
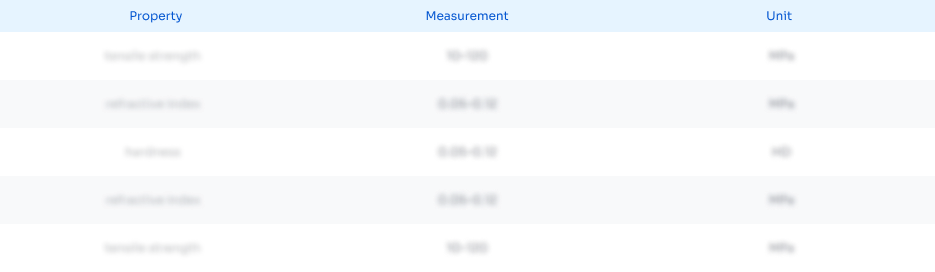
Abstract
Description
Claims
Application Information
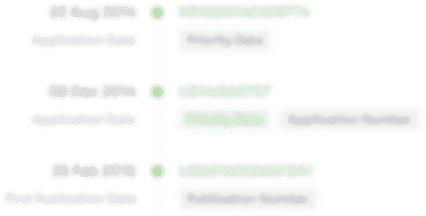
- R&D
- Intellectual Property
- Life Sciences
- Materials
- Tech Scout
- Unparalleled Data Quality
- Higher Quality Content
- 60% Fewer Hallucinations
Browse by: Latest US Patents, China's latest patents, Technical Efficacy Thesaurus, Application Domain, Technology Topic, Popular Technical Reports.
© 2025 PatSnap. All rights reserved.Legal|Privacy policy|Modern Slavery Act Transparency Statement|Sitemap|About US| Contact US: help@patsnap.com