Sunflower disease recognition method based on random forest method
A disease identification and sunflower technology, applied in character and pattern recognition, computer parts, image data processing, etc., can solve problems such as difficult to distinguish new diseases, ambiguity, etc., to improve classification performance, accuracy, and recognition effect. Effect
- Summary
- Abstract
- Description
- Claims
- Application Information
AI Technical Summary
Problems solved by technology
Method used
Image
Examples
Embodiment 1
[0030] Such as figure 1 As shown, the present invention provides a kind of sunflower disease identification method based on random forest method, including four common diseases of sunflower leaves: powdery mildew, bacterial leaf spot, black spot, downy mildew, and as research object:
[0031] A: Disease image collection. The color of the collected leaf image should be as close as possible to the color of the leaf itself.
[0032] B: Disease image preprocessing. Combined with the actual situation, the preprocessing method suitable for sunflower disease image recognition is adopted, the image effect is enhanced by the histogram equalization dehazing algorithm in the airspace, and the weight adaptive image denoising method based on morphology is used to analyze the sunflower leaf disease image. Perform denoising processing.
[0033] C: Lesion image segmentation. After analyzing and comparing various methods of image segmentation, the optimal color image segmentation method is...
Embodiment 2
[0059]In terms of disease spot segmentation: the various models of the color image color space were compared and analyzed. Through comparison, and according to the actual situation of the images collected in Daejeon, the HSV color space, which is sensitive to human vision, was finally selected as the color space for sunflower disease segmentation. By comparing the five image segmentation processing methods, and considering their respective advantages and disadvantages, the K-means clustering algorithm and the watershed algorithm are finally selected to realize the segmentation of color disease images of sunflower leaves and obtain better disease spots. Segment images to provide accurate and good basic images for image feature analysis of sunflower leaf diseases.
[0060] In terms of identification and diagnosis: on the basis of in-depth analysis of disease characteristics, the random forest method is selected to diagnose and identify sunflower diseases. Simulation experiments h...
Embodiment 3
[0069] Disease image segmentation: The purpose of image segmentation is very clear, which is to extract the interesting part of the image for research and processing. The basis is image recognition, and the premise is to identify the disease of the input disease image, which is specifically applied to examples middle. Image segmentation is a key step in the subsequent processing of sunflower disease images, and its accuracy directly has a great impact on disease identification. The key to the image segmentation algorithm is the region in the image, which is divided according to the differences between the regions. Every image segmentation algorithm is not comprehensive, and the segmentation methods may be different for different targets. Therefore, for multiple images with outstanding characteristics, the same method cannot be used only for segmentation. Therefore, the following conditions must be met for each segmentation algorithm: a pixel must only belong to one sub-regio...
PUM
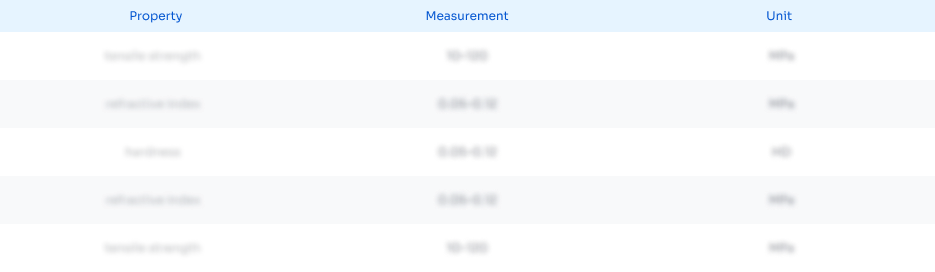
Abstract
Description
Claims
Application Information
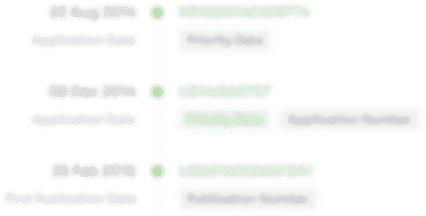
- R&D
- Intellectual Property
- Life Sciences
- Materials
- Tech Scout
- Unparalleled Data Quality
- Higher Quality Content
- 60% Fewer Hallucinations
Browse by: Latest US Patents, China's latest patents, Technical Efficacy Thesaurus, Application Domain, Technology Topic, Popular Technical Reports.
© 2025 PatSnap. All rights reserved.Legal|Privacy policy|Modern Slavery Act Transparency Statement|Sitemap|About US| Contact US: help@patsnap.com