Fan fault detecting method based on depth belief network model
A deep belief network and fault detection technology, applied in wind turbines, engines, mechanical equipment, etc., can solve problems such as long computing time, easy to fall into local optimum, and complex neural network training methods.
- Summary
- Abstract
- Description
- Claims
- Application Information
AI Technical Summary
Problems solved by technology
Method used
Image
Examples
Embodiment
[0115] Select the data for fan status detection. To select the flow rate as the prediction vector, it is necessary to determine the fan parameters related to the fan flow rate as the modeling variables in the observation vector. The Pearson correlation coefficient is used to measure the variable correlation. The Pearson correlation coefficient of each fan status parameter and air volume signal is shown in Table 1.
[0116] Table 1
[0117] parameter name
Pearson correlation coefficient
Fan outlet pressure
0.9592
Fan current (hardwired)
0.8399
Motor coil temperature
0.7351
Motor drive end bearing temperature
0.6467
Motor free end bearing temperature
0.6243
Fan outlet air temperature
0.6169
fan vibration
0.5493
Bearing temperature
0.4827
Fan inlet air temperature
0.4430
Fan oil supply temperature
0.1989
Fan oil tank temperature
0.1004
[0118] Accordin...
PUM
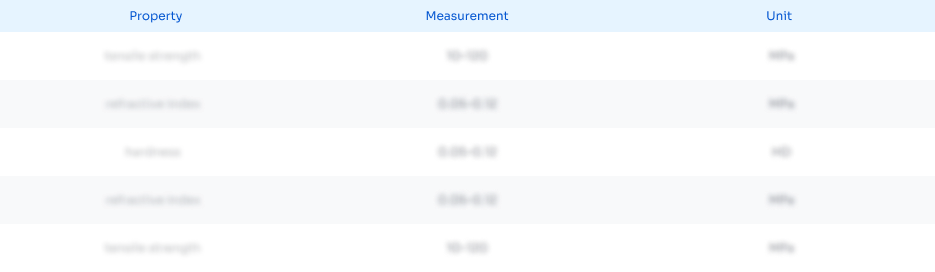
Abstract
Description
Claims
Application Information
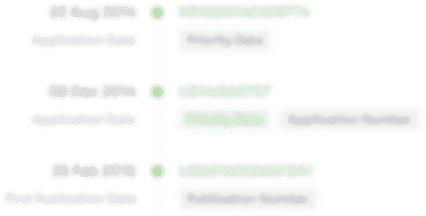
- R&D Engineer
- R&D Manager
- IP Professional
- Industry Leading Data Capabilities
- Powerful AI technology
- Patent DNA Extraction
Browse by: Latest US Patents, China's latest patents, Technical Efficacy Thesaurus, Application Domain, Technology Topic, Popular Technical Reports.
© 2024 PatSnap. All rights reserved.Legal|Privacy policy|Modern Slavery Act Transparency Statement|Sitemap|About US| Contact US: help@patsnap.com