Deep learning algorithm-based classification method of bacterial pneumonia and viral pneumonia in children
A technology of deep learning and classification methods, applied in computing, image analysis, computer components and other directions, it can solve problems such as different images, human bones obscuring human organs, etc., to achieve the effect of reducing the amount of calculation
- Summary
- Abstract
- Description
- Claims
- Application Information
AI Technical Summary
Problems solved by technology
Method used
Image
Examples
Embodiment 1
[0063] Embodiment 1: The specific steps of the classification method of bacterial and viral pneumonia in children based on deep learning algorithm of the present invention
[0064] Such as figure 1 As shown, the classification method of bacterial and viral children's pneumonia based on the deep learning algorithm of the present invention includes (1) in the preprocessing stage, using the full convolutional network semantic segmentation model to carry out transfer learning to segment the lungs from the chest X-ray image region as the region of interest; (2) input the extracted region of interest into the convolutional neural network model to train the classifier to predict the category of the unknown chest X-ray image; (3) use the trained convolutional neural network model to extract The high-dimensional features of the region of interest, while using traditional image processing methods to extract the low-dimensional features of the region of interest, the high and low-dimens...
Embodiment 2
[0089] Embodiment 2: The recognition effect experiment of the classification method of bacterial and viral pneumonia in children based on the deep learning algorithm of the present invention
[0090] 1. Experimental data set: including JSRT public data set (a total of 247 images and segmented lung mask images), Montgomery public data set (a total of 138 images and segmented lung mask images) and women in Guangzhou Children's Hospital dataset (a total of 568 images are divided into two categories: bacterial and viral pneumonia);
[0091] 2. Experimental environment: Matlab 2016a platform, Caffe framework and Python;
[0092] 3. Experimental tool set: full convolutional network model trained by PASCAL VOC2012 dataset, AlexNet convolutional neural network model trained by ImageNet, Anaconda python library;
[0093] 4. Experimental method: The above-mentioned 385 images of JSRT and Montgomery and the lung mask image were divided into a training set and a verification set accordin...
PUM
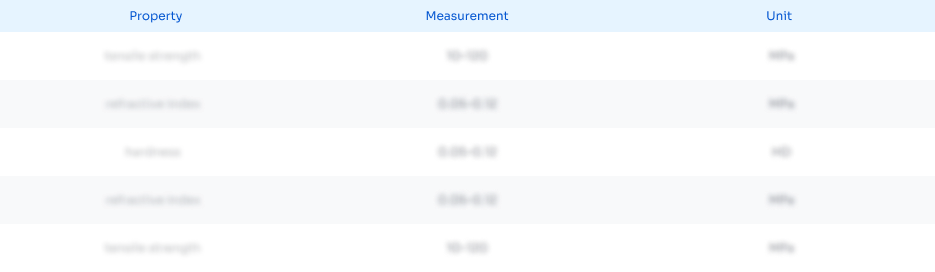
Abstract
Description
Claims
Application Information
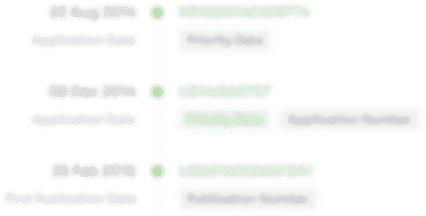
- R&D Engineer
- R&D Manager
- IP Professional
- Industry Leading Data Capabilities
- Powerful AI technology
- Patent DNA Extraction
Browse by: Latest US Patents, China's latest patents, Technical Efficacy Thesaurus, Application Domain, Technology Topic, Popular Technical Reports.
© 2024 PatSnap. All rights reserved.Legal|Privacy policy|Modern Slavery Act Transparency Statement|Sitemap|About US| Contact US: help@patsnap.com