Flow rate level prediction method based on convolutional neural network deep learning
A convolutional neural network and deep learning technology, applied in the field of water regime forecasting in river basins, can solve problems such as increasing the difficulty and complexity of inbound flow forecasting applications, representative influences, etc.
- Summary
- Abstract
- Description
- Claims
- Application Information
AI Technical Summary
Problems solved by technology
Method used
Image
Examples
Embodiment Construction
[0098] The present invention will be further described in detail below in conjunction with the accompanying drawings and specific embodiments to facilitate a clear understanding of the present invention, but they do not limit the present invention.
[0099] Such as figure 1 As shown, the present invention provides a traffic level prediction method based on convolutional neural network deep learning, comprising the following steps:
[0100] (1) Definition of input and output
[0101] 1) Input definition
[0102] Assuming that the influence duration of the previous factors and the future forecast period of a certain reservoir are both 6 hours, there are 130 rainfall stations (numbered 1#-130#) and 16 evaporation stations (numbered 1#-16#) in the control basin of the reservoir area #), 15 soil moisture stations (1#-15# respectively) and 3 meteorological divisions (1#-3# respectively), and there are 2 upper boundary control stations upstream of the reservoir area (1# and 1# resp...
PUM
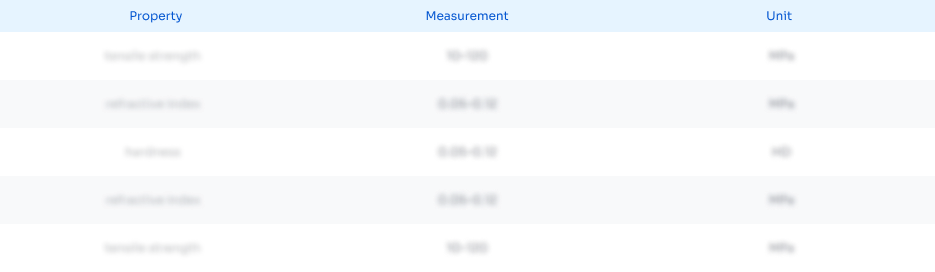
Abstract
Description
Claims
Application Information
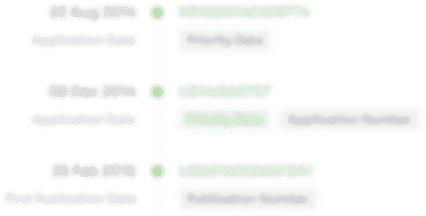
- R&D
- Intellectual Property
- Life Sciences
- Materials
- Tech Scout
- Unparalleled Data Quality
- Higher Quality Content
- 60% Fewer Hallucinations
Browse by: Latest US Patents, China's latest patents, Technical Efficacy Thesaurus, Application Domain, Technology Topic, Popular Technical Reports.
© 2025 PatSnap. All rights reserved.Legal|Privacy policy|Modern Slavery Act Transparency Statement|Sitemap|About US| Contact US: help@patsnap.com