Gear residual life predicting method based on long short-term memory
A technology of long-term and short-term memory and life prediction, which is applied in the testing of machine gears/transmission mechanisms, testing of mechanical components, testing of machine/structural components, etc. Explosion, gradient dissipation and other problems
- Summary
- Abstract
- Description
- Claims
- Application Information
AI Technical Summary
Problems solved by technology
Method used
Image
Examples
Embodiment Construction
[0131] In order to make the objects, features and advantages of the present invention more comprehensible, specific implementations of the present invention will be described in detail below in conjunction with the accompanying drawings.
[0132] In the embodiment of the present invention, the real-time remaining life prediction method of gears, the method flow chart is as follows figure 1 Shown: Include the following steps:
[0133] Step 1. Carry out fatigue tests on gears to obtain real-time monitoring data that characterizes gear degradation:
[0134] The gear fatigue life test uses a power flow closed test bench. The center distance of the test bench is 150mm, and the motor speed is 1200r / min. During the test, the vibration of the box, oil temperature and noise are monitored. In the test, the material is alloy steel, the tooth surface hardness is 58-61HRC hard tooth surface gear, and the surface treatment is carburizing and quenching. The front and back sides are stagg...
PUM
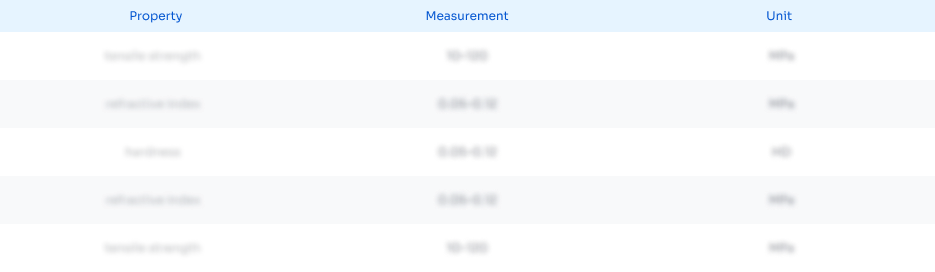
Abstract
Description
Claims
Application Information
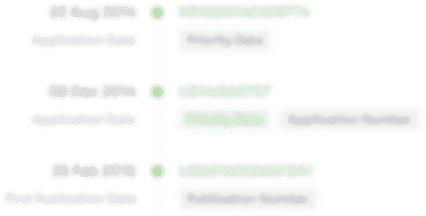
- R&D
- Intellectual Property
- Life Sciences
- Materials
- Tech Scout
- Unparalleled Data Quality
- Higher Quality Content
- 60% Fewer Hallucinations
Browse by: Latest US Patents, China's latest patents, Technical Efficacy Thesaurus, Application Domain, Technology Topic, Popular Technical Reports.
© 2025 PatSnap. All rights reserved.Legal|Privacy policy|Modern Slavery Act Transparency Statement|Sitemap|About US| Contact US: help@patsnap.com