Speech recognition model establishing method based on bottleneck characteristics and multi-scale and multi-headed attention mechanism
A technology of speech recognition model and establishment method, applied in the field of training model, can solve the problems of single attention scale and poor recognition performance, achieve powerful time series modeling ability and distinguish ability, improve accuracy, and improve the effect of recognition effect
- Summary
- Abstract
- Description
- Claims
- Application Information
AI Technical Summary
Problems solved by technology
Method used
Image
Examples
specific Embodiment approach 1
[0018] The method for establishing a speech recognition model based on bottleneck features and a multi-scale multi-head attention mechanism of the present embodiment, the method includes the following steps:
[0019] Step 1, utilize the input sample FBank speech feature vector X=(x 1 ,x 2 ,...,x T ) to perform unsupervised training on the RBM network in the DBN, and obtain the connection weight matrix W of the first three layers in the initialization coding network 1 , W 2 , W 3 , the weight matrix connected by these three layers and a layer of randomly initialized weight output layer W 4 The bottleneck feature extraction network based on DBN that constitutes the front end of the encoding network; RBM network means restricted Boltzmann machine, the English full name is Restricted Boltzmann Machine, referred to as RBM; DBN means deep belief network, English full name is Deep Belief Network, referred to as DBN; FBank represents a filter bank; Sample FBank speech feature vec...
specific Embodiment approach 2
[0027] Different from the specific embodiment one, the speech recognition model establishment method based on the bottleneck feature and the multi-scale multi-head attention mechanism of the present embodiment, in the described step one, the input speech feature vector X=(x 1 ,x 2 ,...,x T ) using 40-dimensional FBank features and energy, and then stitching corresponding to the first-order and second-order differences, a total of 123-dimensional parameters; for the extracted features, first normalize within the scope of the training set, so that each component obeys the standard normal distribution, Then use the normalization parameters of the training set to normalize the features of the test set and development set.
specific Embodiment approach 3
[0029] Different from the specific embodiment one or two, the speech recognition model establishment method based on the bottleneck feature and the multi-scale multi-head attention mechanism of the present embodiment, in the described step one and step two, the RBM network in the DBN is wirelessly Supervised training process, wherein, the training of RBN network comprises adopting unsupervised pre-training (pretraining) and reverse gradient propagation algorithm to have supervised training method; The input of described RBM network is FBank speech feature, and the output layer of RBM network is softmax layer , each output layer unit corresponds to the posterior probability of the bound triphone state; there are three hidden layers between the input layer and the output layer, the second hidden layer is the bottleneck layer, and the state of the second hidden layer The number of units is less than other hidden layers.
PUM
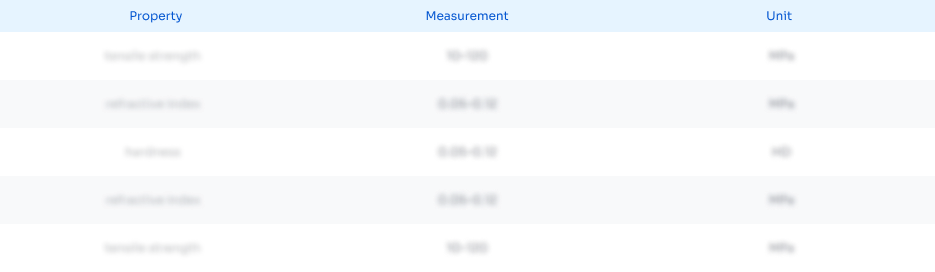
Abstract
Description
Claims
Application Information
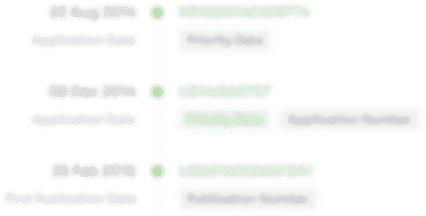
- R&D
- Intellectual Property
- Life Sciences
- Materials
- Tech Scout
- Unparalleled Data Quality
- Higher Quality Content
- 60% Fewer Hallucinations
Browse by: Latest US Patents, China's latest patents, Technical Efficacy Thesaurus, Application Domain, Technology Topic, Popular Technical Reports.
© 2025 PatSnap. All rights reserved.Legal|Privacy policy|Modern Slavery Act Transparency Statement|Sitemap|About US| Contact US: help@patsnap.com