Nonlinear equalization method based on gated recurrent neural network
A technology of cyclic neural network and equalization method, which is applied in the field of nonlinear equalization based on gated cyclic neural network, can solve problems such as large complexity, dependence, and application limitations, and achieve high-performance nonlinear loss compensation and low nonlinearity Loss Compensation, Effects of High-Performance Complexity
- Summary
- Abstract
- Description
- Claims
- Application Information
AI Technical Summary
Problems solved by technology
Method used
Image
Examples
Embodiment Construction
[0024] In order to make the object, technical solution and advantages of the present invention clearer, the present invention will be described in further detail below in conjunction with specific embodiments and with reference to the accompanying drawings.
[0025] It should be noted that all expressions using "first" and "second" in the embodiments of the present invention are to distinguish two entities with the same name but different parameters or parameters that are not the same, see "first" and "second" It is only for the convenience of expression, and should not be construed as a limitation on the embodiments of the present invention, which will not be described one by one in the subsequent embodiments.
[0026] figure 1 It is a schematic flow chart of the method of the embodiment of the present invention. As shown in the figure, the non-linear equalization method based on the gated recurrent neural network provided by the embodiment of the present invention includes:...
PUM
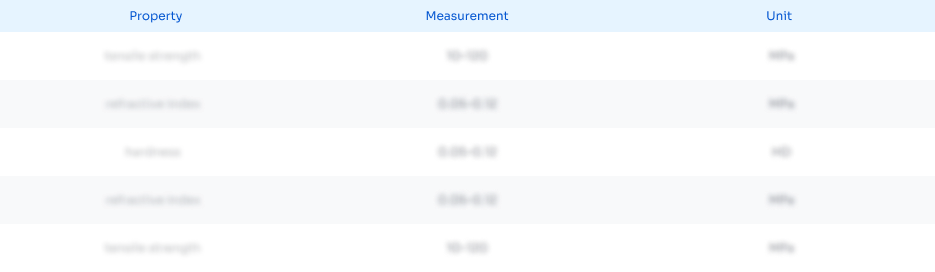
Abstract
Description
Claims
Application Information
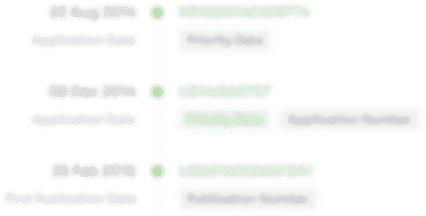
- R&D Engineer
- R&D Manager
- IP Professional
- Industry Leading Data Capabilities
- Powerful AI technology
- Patent DNA Extraction
Browse by: Latest US Patents, China's latest patents, Technical Efficacy Thesaurus, Application Domain, Technology Topic, Popular Technical Reports.
© 2024 PatSnap. All rights reserved.Legal|Privacy policy|Modern Slavery Act Transparency Statement|Sitemap|About US| Contact US: help@patsnap.com