Motor bearing fault diagnosis method
A fault diagnosis and motor bearing technology, applied in mechanical bearing testing, neural learning methods, computer components, etc., can solve problems such as the influence of equipment operating data, algorithm difficulty, and no diagnostic model design, so as to alleviate the problem of model overfitting , Improve the training speed and accuracy, and improve the effect of diagnosis and recognition rate
- Summary
- Abstract
- Description
- Claims
- Application Information
AI Technical Summary
Problems solved by technology
Method used
Image
Examples
Embodiment 1
[0056] The public motor bearing data set provided by the laboratory of Western Reserve University is used as the training set. The data set includes the motor normal bearing data, the drive end bearing fault data and the fan end bearing fault data, and the bearing fault location includes the rolling element fault, the inner ring Fault and outer ring fault, the fault size is 0.007, 0.014, 0.021 and 0.028 inch four different damage diameters to simulate different fault degrees of the bearing; the motor speed is 1797, 1772, 1750 and 1730r / min, corresponding to 0, 1 , 2, and 3hp loads, the sampling frequency is 12kHz; the length of a single sample is 400, the update ratio of the discriminative model and the generative model is 1:2, and the learning rates of the generative model and the confrontation model are 0.0001 and 0.00001, respectively. Batches of 10 samples are read.
[0057] Such as Figure 5 As shown, after 5400 times of training, the final loss value remains at about 0....
PUM
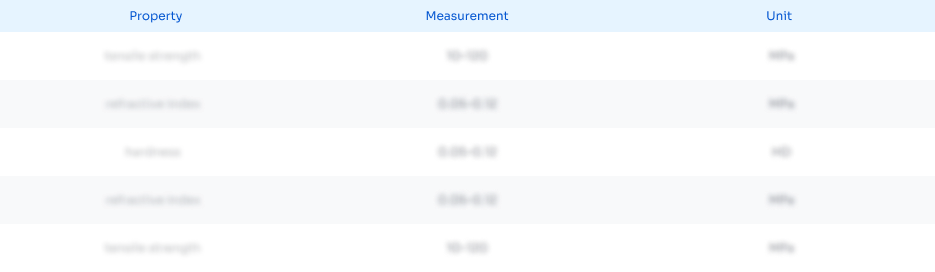
Abstract
Description
Claims
Application Information
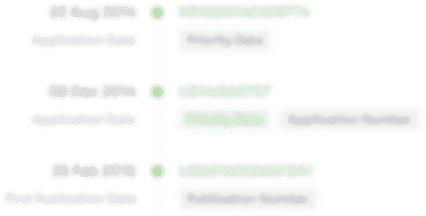
- R&D Engineer
- R&D Manager
- IP Professional
- Industry Leading Data Capabilities
- Powerful AI technology
- Patent DNA Extraction
Browse by: Latest US Patents, China's latest patents, Technical Efficacy Thesaurus, Application Domain, Technology Topic, Popular Technical Reports.
© 2024 PatSnap. All rights reserved.Legal|Privacy policy|Modern Slavery Act Transparency Statement|Sitemap|About US| Contact US: help@patsnap.com